A lot of interest has gone into conveniently and quickly detecting facial gestures of users lately using low quality image feeds. I am going to stab at doing this in Mathematica here., Check out the youtube video for a walk-through:
Facial Gesture Detection using Mathematica 10
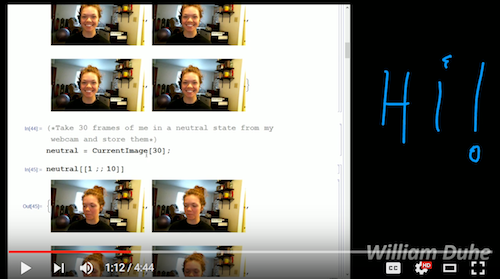
I will take a series of images from our connected webcam and then train a machine learning process to identify specific facial gestures based on the images. The proper way to control how fast one can capture images from the camera is with the Device property "FrameRate". My understanding is that this controls the frequency that the ScheduledTask runs at, I found that in testing trying to run this little demo with just one person in the camera's viewpoint caps out in performance at around 15 FPS.
camera = DeviceOpen["Camera"];
camera["FrameRate"] = 15;
camera["RasterSize"] = {480, 340};
camera["Timeout"] = 900;
Take 30 frames of me smiling from my webcam and store them - then show the first 10 images.
smiles = CurrentImage[30];
smiles[[1 ;; 10]]
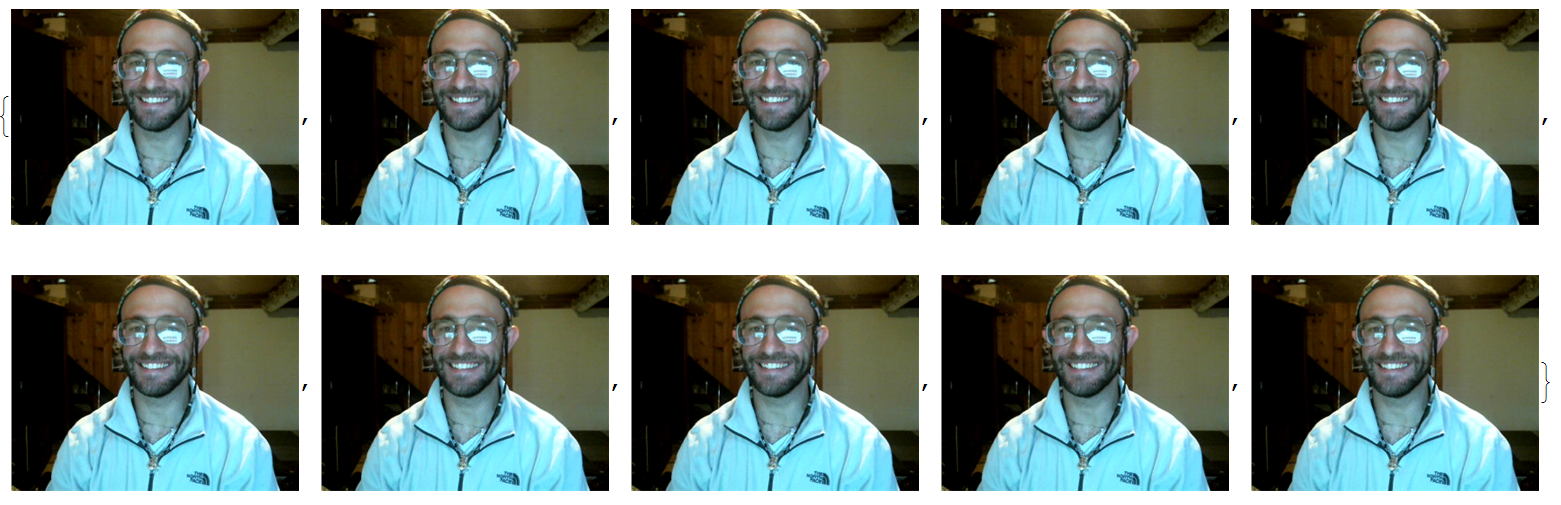
Do the same thing for a neutral facial state
neutral = CurrentImage[30];
neutral[[1 ;; 10]]
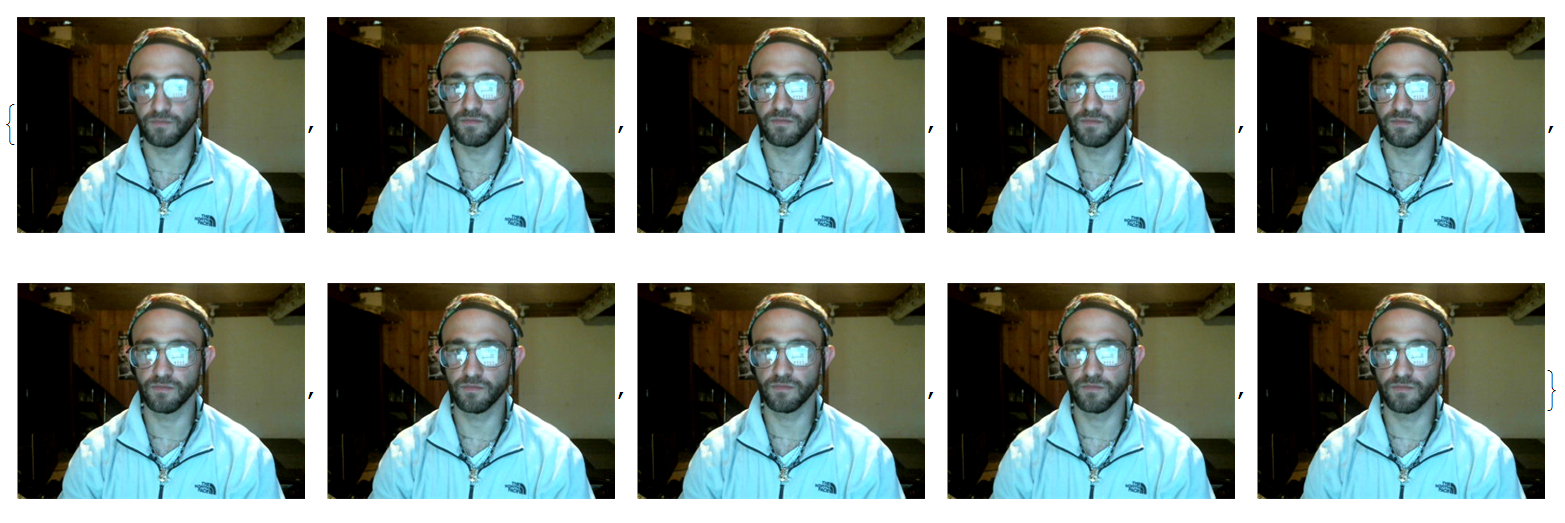
This function takes a list of elements and associates them with a feature
createAssociation[dataSet_, feature_] :=
Table[
dataSet[[i]] -> feature,
{i, 1, Length[dataSet]}]
Here we have a function that trims the faces out of a set of images for analysis
takeFaces[dataSet_] :=
(
Module[{tmpVar},
tmpVar = Table[
(ImageTrim[dataSet[[i]], #] & /@
FindFaces[dataSet[[i]](*,{50,150}*)])[[1]],
{i, 1, Length[dataSet]}];
Select[tmpVar, UnsameQ[#, {}] &]
]
)
Now create two separate training sets which are associations from the gathered images. We also trim the faces from these images. Show the first ten elements of both generated data sets.
smileData = createAssociation[takeFaces[smiles], "Smile"];
neutralData = createAssociation[takeFaces[neutral], "Neutral"];
smileData[[1 ;; 10]]
neutralData[[1 ;; 10]]
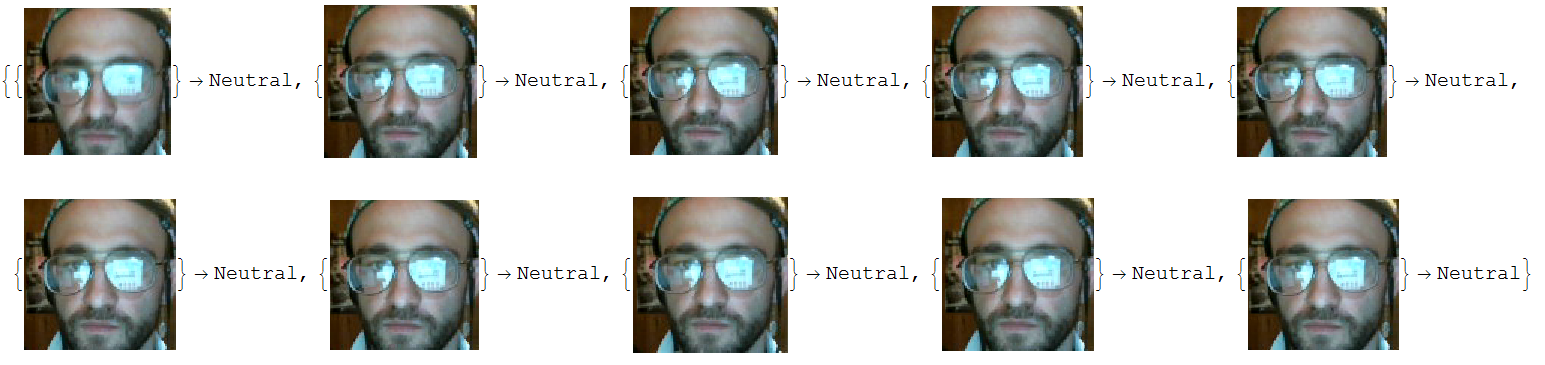
Create a classifier from the gathered images setting the method to quality
c1 = Classify[Join[smileData, neutralData], Method -> "NeuralNetwork",
PerformanceGoal -> "Quality"]
This is the main function that labels faces in the image. We use fold to apply ImageCompose to the original image, nesting the ImageComposes like so ImageCompose[ImageCompose[img, label1], label2]. This allows us full support for as many faces as necessary in the future, not just one. You can edit the styling options here if you want the text to be styled differently, I didn' t generalize out those options but that part of the function is pretty easily modified
imageLabel[image_, labelFunc_] :=
Module[{img = image, faces},
faces = FindFaces[img];
If[Flatten[faces] === {}, img,
Fold[ImageCompose[#1, Sequence @@ #2] &,
HighlightImage[img, Join[fullRectangleInLines /@ faces],
"HighlightColor" ->
Blue], {Graphics[
Text[Style[labelFunc[ImageTrim[img, #]], Black, Bold, Italic,
16, Background -> White]]], (First[#] + {(
First[Last[#]] - First[First[#]])/2, -25})} & /@ faces]]]
This is a helper function that takes the coords produced by FindFaces and makes 4 line objects for the box around the face.
fullRectangleInLines[positions_] :=
Line[{
{First[First[positions]], Last[First[positions]]},
{First[First[positions]], Last[Last[positions]]},
{First[Last[positions]], Last[Last[positions]]},
{First[Last[positions]], Last[First[positions]]},
{First[First[positions]], Last[First[positions]]}
}]
This is just a simple labeling function that returns a string of a number that gets incremented every time it is run.It doubles as a useful little framerate function too in the dynamic version.
labelingFunc[i_Image] :=
Column[{
"User: William Duhe",
"Gesture: " <>
c1[ImageTrim[CurrentImage[], #] & /@
FindFaces[CurrentImage[], {50, 150}]]
}]
Here I take a single frame from from the feed which shows you the facial gesture of the user bellow it. I do this for both smiling and neutral as a test.
imageLabel[CurrentImage[], labelingFunc]
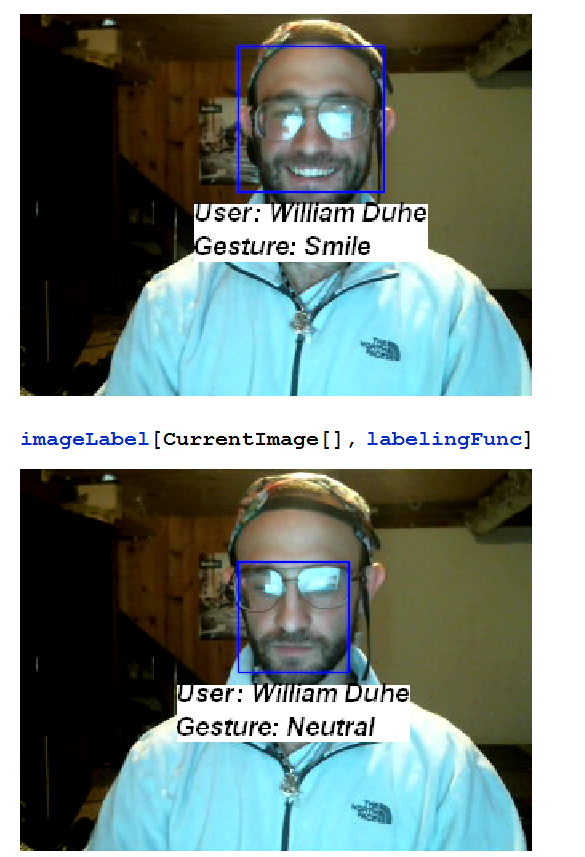
Thanks for taking the time to read through this post and I hope that you find it useful! I might make another post where I wrap all this up in a nice toolkit which allows you to train different states using buttons and then show a live stream of the webcam labeling the users gestures using a Dynamically updating feed. Let me know if anyone would find this useful.