Recognizing Kiwi Bird Calls in Audio Recordings
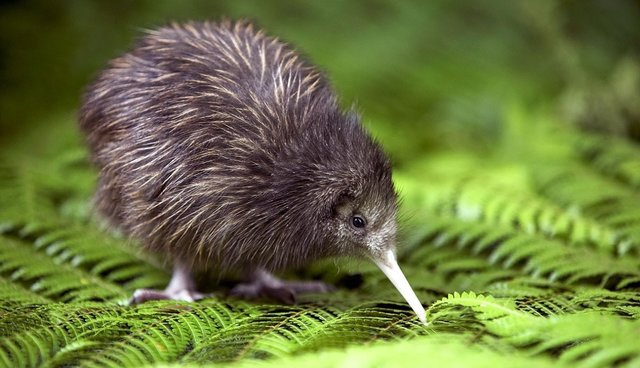
My project for WSC 2017 was identifying kiwi calls in audio recordings. The project can be broken down into 2 main steps:
- Finding clips that contain noise that could be a kiwi
- Identifying the clips that actually contain a kiwi call
Finding Clips
Data
The data is an audio recording taken overnight in Northland, New Zealand
Filtering
I started off by filtering the audio to be between 1200Hz and 3600Hz in order to remove the majority of the noise. I then normalized the audio to make the volume consistent.
AudioNormalize[HighpassFilter[LowpassFilter[audio, Quantity[1200, "Hertz"]], Quantity[3600, "Hertz"]]]
At first I just took any intervals of audio that were above a certain threshold.
AudioIntervals[audioProcessed, #RMSAmplitude > 0.02 &]
This yielded many very short clips so I then extended each clip 1 second in each direction and merged clips that were within 2 seconds of each other.
({#[[1]] - 1, #[[2]] + 1} & /@ AudioIntervals[audioProcessed, #RMSAmplitude > 0.02 &])
//. {pre___, {c_, d_}, {x_, y_}, post___} /; d > x - 2 -> {pre, {c, y}, post}
This still had the problem of containing many clips just over 2 seconds long so I removed any clips less than 10 seconds as kiwi calls are longer than ten seconds.
Cases[int, {x_, y_} /; y - x > 10]
I then limited the length of each of the clips to exactly 10 seconds to make it easier for the machine learning.
AudioTrim[#,10]&/@clips
Identifying calls
Unsupervised Learning
Since my data was unclassified I initially tried to use unsupervised learning for clustering the audio clips, although this didn't yield any particularly meaningful results.
FeatureSpacePlot[clips, LabelingFunction -> (#2[[2]] &), PerformanceGoal -> "Quality"]
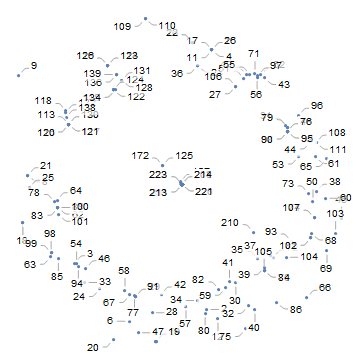
The middle section is about 50% kiwi calls and 50% not kiwi calls, as is the circle around the outside so I have no idea what the feature extractor is looking at.
Classifying Data
Since unsupervised learning didn't work particularly well I decided to manually classify every single clip.
Doesn't that sound fun...
clipId = 1;
clipClasses = Range@Length[clips2];
Dynamic@Row[{clipId, "/", Length[clips2],
If[clipId <= Length[clips2], clips2[[clipId]], "DONE!"] k,
Button["Yes", clipClasses[[clipId]] = "Kiwi"; clipId = clipId + 1],
Button["No", clipClasses[[clipId]] = "NotKiwi";
clipId = clipId + 1]}]
Dynamic[Row[{clipId - 1, clipClasses[[clipId - 1]]}]]
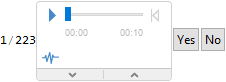
Neural Network - Take One
I used 200 of the clips as training data for the neural network.
data=Thread[clips,clipClasses];
training=RandomSample[data,200];
Counts[training]
test=Complement[data,training];
Counts[test]
I used a neural net to classify the audio clips as it was the best at classifying them.
cf=Classify[training,Method->"NeuralNetwork",PerformanceGoal->"Quality"]
cm=ClassifierMeasurements[cf,test]
The accuracy of the neural network was extremely poor though
cm["Accuracy"]
0.559565
Neural Network - Take Two
I then tried downsampling the audio from 44.1kHz to 10kHz to reduce the amount of extraneous data the neural network has to work with.
clipsSmall = AudioResample[#, Quantity[10, "Kilohertz"]] & /@ clips;
This reduced the amount of data without significantly changing the audio
cm["Accuracy"]
0.453762
Well back to the drawing board I guess
Neural Network - Take Three
This time I tried a different approach, since the neural network seemed to handle audio extremely poorly I instead input the spectrogram of the audio into the neural net.
data= Thread[Image[Abs[SpectrogramArray[#]]] & /@ clipsSmall -> clipClasses[[All, 1]]]
The image processing side has had a lot more work done so this should work much better.
cm["Accuracy"]
0.820756
At this point I ran out of ways to improve the score and ran up against the limit of the accuracy that I was able to classify the clips so I'm going to call that a success.
Finding calls
Finally finding the calls, which is the simple now that we can find and classify potential calls
FindCalls[clip_] := (Module[{int = {}, audioProcessed, clips, classes},
audioProcessed = ProcessAudio[clip];
int = ProcessAudioIntervals[audioProcessed];
int = Cases[int, {x_?NumberQ, y_?NumberQ} /; y - x >= 10];
clips = Which[Length[int] == 0, {},
Length[int] == 1,
AudioTrim[#, 10] & /@ {AudioTrim[audioProcessed, int]},
Length[int] > 1,
AudioTrim[#, 10] & /@ AudioTrim[audioProcessed, int]];
classes =
KiwiCallClassifier[
Image[Abs[
SpectrogramArray[
AudioResample[#, Quantity[10, "Kilohertz"]]]]] & /@ clips];
Thread[{Extract[clips, Position[classes, "Kiwi"]],
Quantity[#, "Seconds"] & /@
Extract[int, Position[classes, "Kiwi"]][[All, 1]]}]])
FindCalls[Import@"C:\\Users\\Isaac\\Desktop\\Programming\\data\\Kiwi Audio\\Processing\\20170604k-53.mp3"] // Grid
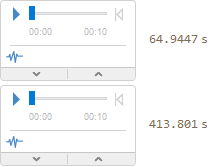
Reflections
Good
Actually finding clips with noise was quite a simple and easy task, I just messed around with the frequencies on the filters and the threshold for a while.
Bad
In hindsight I realize that this wasn't a problem that was particularly suited to unsupervised learning, as there are other more general features than "kiwi" or "not kiwi" for a feature extractor to identify (although I still have no idea what it was doing).
Worst
Another thing that's important for undertaking a machine learning project like this is having a lot of data. If I do another project like this I'm definitely going to use more, already classified data instead of spending 2 hours listening to birds screaming and loud backgound noise.
This is the training data I used for the neural net https://drive.google.com/file/d/0B4VdlZ57AG6BcXBMMi1NQnQyRms/view?usp=sharing