We aim to create a music genre classifier which allows the detection of the genre of audio/music files. The dataset used for training the model is the GTZAN dataset, it consists of 1000 audio tracks each 30 seconds long. It contains 10 genres, each of them have 100 tracks. For feature extraction, we will be extracting the MFCC values of the audio file. MFCC's are commonly used as features in speech recognition and music information retrieval systems.
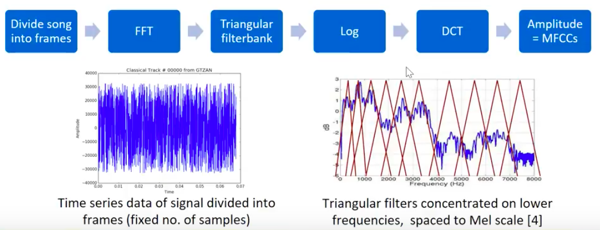
We divided each song into two parts of 15 seconds each, this way we get more data and our dataset increases to 2000 songs. We will be extracting the MFCC values of all the audio files by partitioning the song into 15 seconds each.
In[25]:= rockdata =
Import["/Users/aishwaryapraveen/Desktop/Summer School \
Project/genres/rock/*.au"];
rockdata1 = Flatten[AudioSplit[#, 15] & /@ rockdata];
countrydata =
Import["/Users/aishwaryapraveen/Desktop/Summer School \
Project/genres/country/*.au"];
In[29]:= countrydata1 = Flatten[AudioSplit[#, 15] & /@ countrydata];
In[30]:= bluesdata =
Import["/Users/aishwaryapraveen/Desktop/Summer School \
Project/genres/blues/*.au"];
bluesdata1 = Flatten[AudioSplit[#, 15] & /@ bluesdata];
In[33]:= classicaldata =
Import["/Users/aishwaryapraveen/Desktop/Summer School \
Project/genres/classical/*.au"];
In[34]:= classicaldata1 =
Flatten[AudioSplit[#, 15] & /@ classicaldata];
In[10]:= discodata =
Import["/Users/aishwaryapraveen/Desktop/Summer School \
Project/genres/disco/*.au"];
In[35]:= discodata1 = Flatten[AudioSplit[#, 15] & /@ discodata];
In[36]:= Length@discodata1
Out[36]= 200
In[39]:= hiphopdata =
Import["/Users/aishwaryapraveen/Desktop/Summer School \
Project/genres/hiphop/*.au"];
In[38]:= hiphopdata1 = Flatten[AudioSplit[#, 15] & /@ hiphopdata];
In[40]:= jazzdata =
Import["/Users/aishwaryapraveen/Desktop/Summer School \
Project/genres/jazz/*.au"];
jazzdata1 = Flatten[AudioSplit[#, 15] & /@ jazzdata];
In[42]:= metaldata =
Import["/Users/aishwaryapraveen/Desktop/Summer School \
Project/genres/metal/*.au"];
metaldata1 = Flatten[AudioSplit[#, 15] & /@ metaldata];
In[130]:=
popdata =
Import["/Users/aishwaryapraveen/Desktop/Summer School \
Project/genres/pop/*.au"];
In[131]:= popdata1 = Flatten[AudioSplit[#, 15] & /@ popdata];
In[46]:= reggaedata =
Import["/Users/aishwaryapraveen/Desktop/Summer School \
Project/genres/reggae/*.au"];
In[47]:= reggaedata1 = Flatten[AudioSplit[#, 15] & /@ reggaedata];
MFCC Extraction
mFCCFeaturesreggaedata = (Values@
AudioLocalMeasurements[#, "MFCC",
PartitionGranularity -> {1., 1.}]) & /@ reggaedata1;
mFCCFeaturesClassReggae = Thread[mFCCFeaturesreggaedata -> "reggae"];
mFCCFeaturespopdata = (Values@
AudioLocalMeasurements[#, "MFCC",
PartitionGranularity -> {1., 1.}]) & /@ popdata1;
mFCCFeaturesClassPop = Thread[mFCCFeaturespopdata -> "pop"];
mFCCFeaturesmetaldata = (Values@
AudioLocalMeasurements[#, "MFCC",
PartitionGranularity -> {1., 1.}]) & /@ metaldata1;
mFCCFeaturesClassMetal = Thread[mFCCFeaturesmetaldata -> "metal"];
mFCCFeaturesjazzdata = (Values@
AudioLocalMeasurements[#, "MFCC",
PartitionGranularity -> {1., 1.}]) & /@ jazzdata1;
mFCCFeaturesClassJazz = Thread[mFCCFeaturesjazzdata -> "jazz"];
mFCCFeatureshiphopdata = (Values@
AudioLocalMeasurements[#, "MFCC",
PartitionGranularity -> {1., 1.}]) & /@ hiphopdata1;
mFCCFeaturesClasshiphop = Thread[mFCCFeatureshiphopdata -> "hiphop"];
mFCCFeaturesdiscodata = (Values@
AudioLocalMeasurements[#, "MFCC",
PartitionGranularity -> {1., 1.}]) & /@ discodata1;
mFCCFeaturesClassdisco = Thread[mFCCFeaturesdiscodata -> "disco"];
mFCCFeaturesbluesdata = (Values@
AudioLocalMeasurements[#, "MFCC",
PartitionGranularity -> {1., 1.}]) & /@ bluesdata1;
mFCCFeaturesClassblues = Thread[mFCCFeaturesbluesdata -> "blues"];
mFCCFeaturesclassicaldata = (Values@
AudioLocalMeasurements[#, "MFCC",
PartitionGranularity -> {1., 1.}]) & /@ classicaldata1;
mFCCFeaturesClassclassical =
Thread[mFCCFeaturesclassicaldata -> "classical"];
mFCCFeaturesrockdata = (Values@
AudioLocalMeasurements[#, "MFCC",
PartitionGranularity -> {1., 1.}]) & /@ rockdata1;
mFCCFeaturesClassrock = Thread[mFCCFeaturesrockdata -> "rock"];
mFCCFeaturescountrydata = (Values@
AudioLocalMeasurements[#, "MFCC",
PartitionGranularity -> {1., 1.}]) & /@ countrydata1;
mFCCFeaturesClasscountry =
Thread[mFCCFeaturescountrydata -> "country"];
First we will be implementing a neural network only on the first three genres to see how it performs, our training set consists of 540 songs and the validation set consists of 60 songs.
net = NetChain[{
GatedRecurrentLayer[128],
GatedRecurrentLayer[128],
SequenceLastLayer[],
LinearLayer[],
SoftmaxLayer[]},
"Input" -> {"Varying", 13},
"Output" -> NetDecoder[{"Class", {"metal", "pop", "reggae"}}]]
data = RandomSample[
Join[mFCCFeaturesClassPop, mFCCFeaturesClassReggae,
mFCCFeaturesClassMetal]];
trainSet1 = data[[1 ;; 540]];
validationSet1 = data[[541 ;;]];
trainedNet =
NetTrain[net, trainSet1, ValidationSet -> validationSet1,
MaxTrainingRounds -> 100]
cl5 = ClassifierMeasurements[trainedNet, validationSet1]
In[582]:= cl5["Accuracy"]
Out[582]= 0.733333
Confusion Matrix Plot 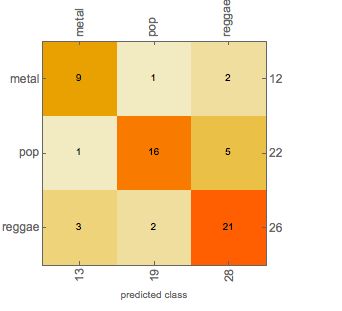
We implement a different architecture of the recurrent neural network for all the 10 genres, this time our training set is 1900 songs and validation set is 100 songs.
net4 = NetChain[{
GatedRecurrentLayer[256],
GatedRecurrentLayer[256],
GatedRecurrentLayer[256],
SequenceLastLayer[],
LinearLayer[],
SoftmaxLayer[]},
"Input" -> {"Varying", 13},
"Output" ->
NetDecoder[{"Class", {"country", "blues", "disco", "hiphop",
"jazz", "metal", "pop", "reggae", "rock", "classical"}}]
]
data = RandomSample[
Join[mFCCFeaturesClassPop, mFCCFeaturesClassReggae,
mFCCFeaturesClassMetal, mFCCFeaturesClassJazz,
mFCCFeaturesClassblues, mFCCFeaturesClassclassical,
mFCCFeaturesClasscountry, mFCCFeaturesClassdisco,
mFCCFeaturesClassrock, mFCCFeaturesClasshiphop]];
trainSet = data[[1 ;; 1900]];
validationSet = data[[1901 ;;]];
trainednet4 =
NetTrain[net4, trainSet, ValidationSet -> validationSet,
MaxTrainingRounds -> 100]
cl = ClassifierMeasurements[trainednet4, validationSet]
In[620]:= cl["Accuracy"]
Out[620]= 0.75
Confusion Matrix Plot
We achieve an accuracy of 75% for classifying the genres of the audio files.
We will now construct a function which takes in an audio and classifies it into a genre.
In[571]:=
findGenre[sound_] :=
With[{audio =
Values@AudioLocalMeasurements[AudioResample[sound, 22050], "MFCC",
PartitionGranularity -> {1., 1.}]},
trainednet4[audio]
]
In[577]:= findGenre[rockdata[[-3]]]
Out[577]= "rock"
In[587]:= findGenre[countrydata[[-2]]]
Out[587]= "country"