Well, the variance does increase away from the data, just maybe not as quickly as you'd expect. From what I understood, this depends on the details of your network (the activation function, in particular) and the training parameters. Ultimately, those factors decide what kind of Gaussian process kernel you're effectively using, but the connection between the two isn't straightforward (and an active area of research, as far as I could figure out).
Also, Gaussian processes do not necessarily produce arbitrarily large variance away from the data from what I've seen. If you take a simple example from the documentation of Predict
, you can quite easily get a constant error band away from the data:
data = {-1.2 -> 1.2, 1.4 -> 1.4, 3.1 -> 1.8, 4.5 -> 1.6}; p =
Predict[data, Method -> "GaussianProcess"];
Show[Plot[{p[x],
p[x] + StandardDeviation[p[x, "Distribution"]],
p[x] - StandardDeviation[p[x, "Distribution"]]},
{x, -5, 10},
PlotStyle -> {Blue, Gray, Gray},
Filling -> {2 -> {3}},
Exclusions -> False,
PerformanceGoal -> "Speed",
PlotLegends -> {"Prediction", "Confidence Interval"}],
ListPlot[List @@@ data, PlotStyle -> Red, PlotLegends -> {"Data"}]]
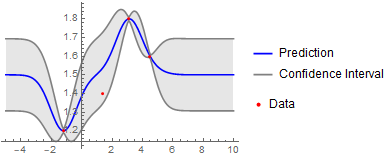