This is the second part of an ongoing series. The first part can be found here . I have since improved the code from the first part with a neural network and use of the new SequencePredict function. At the end of this post is all the new code from Part 1 and Part 2.
Poems often rhyme. A naive explanation of rhyme says that one word rhymes with another if the ending sounds are the same. Rhyme in context is more complicated than that, and the concept of rhyme can be extended to a larger class of what we might call sound echoes. Inside a poem, we rhyme one part of the stress pattern with another, a stressed syllable followed by zero to two unstressed syllables. With this definition, we can understand rhymes like "...pick it..." with "thicket" and "Lenore" with "nevermore". The term rhyme can include a larger family of sound features in a poem. Here, we will consider traditional rhyme (end rhyme), alliteration (front rhyme), assonance (vowel rhyme), and consonance (consonant rhyme). Poets are certainly aware of these echoic features, often plying them for effect. With the help of the Wolfram Language, we can represent these rhymes in an informative way.
Alliteration
To map the rhymes across an image of the stanza, I need four pieces of information: the phonetic representation of each word, each word broken into separate syllables, the phonetic representation of each syllable, and the stress of each syllable. With thoughtful application of Wolfram magic, alliteration looks like this:
Some alliterations ring louder than others, and we can analyze that too. If the alliteration is between two stressed syllables, then we hear the echo clearly. If not, then the echo is faint. This could be represented by line thickness or transparency, though I have not yet implemented this idea. You might also notice that the computer is not always interpreting the sound pairs correctly. For instance, it says that the second syllable of weary alliterates with the word while, which is certainly not true. The algorithm I am using to get the phonetics of the syllables has room for improvement.
Consonance
For consonance, which is the repetition of consonant sounds anywhere in two syllables, we change the code slightly. 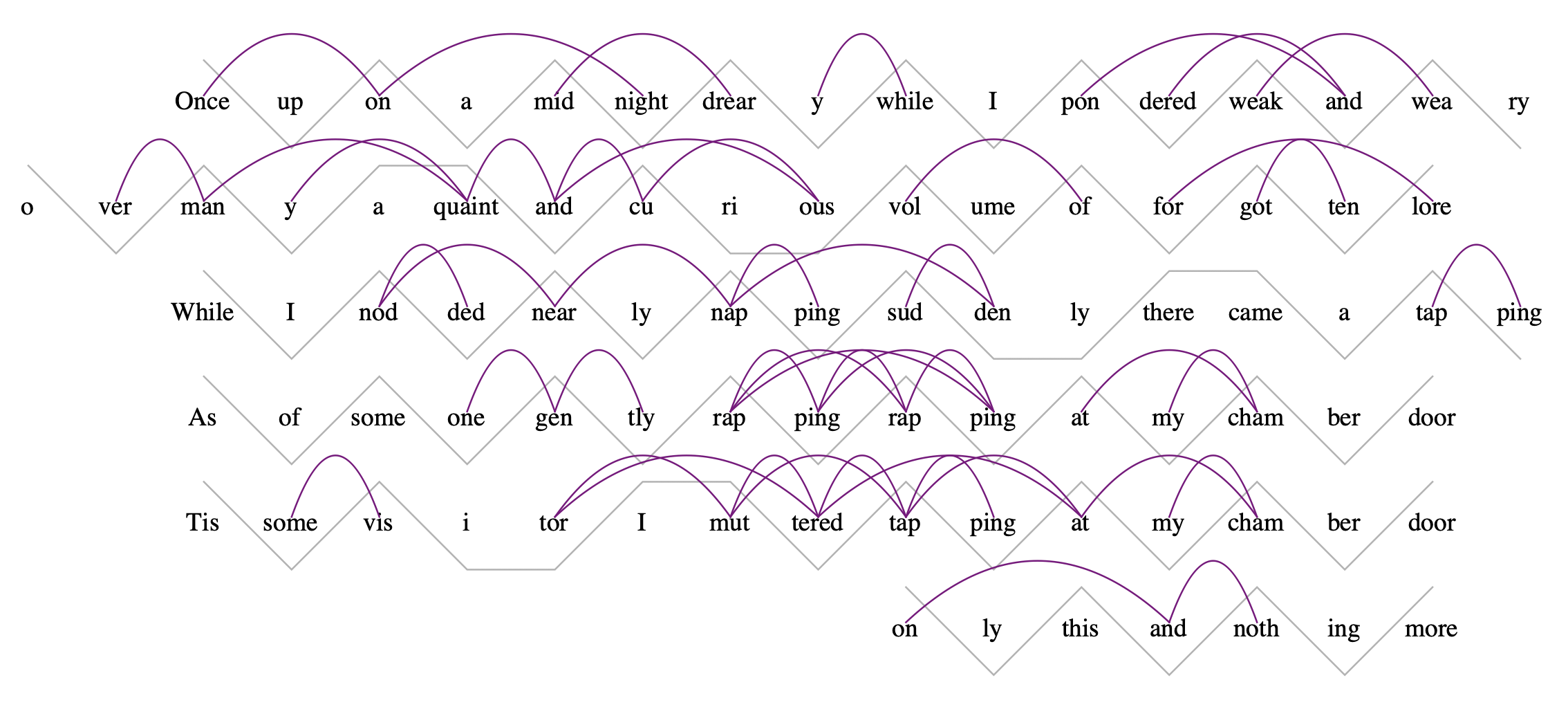
Assonance
And again for assonance, the echo of vowel sounds, the code is slightly different. 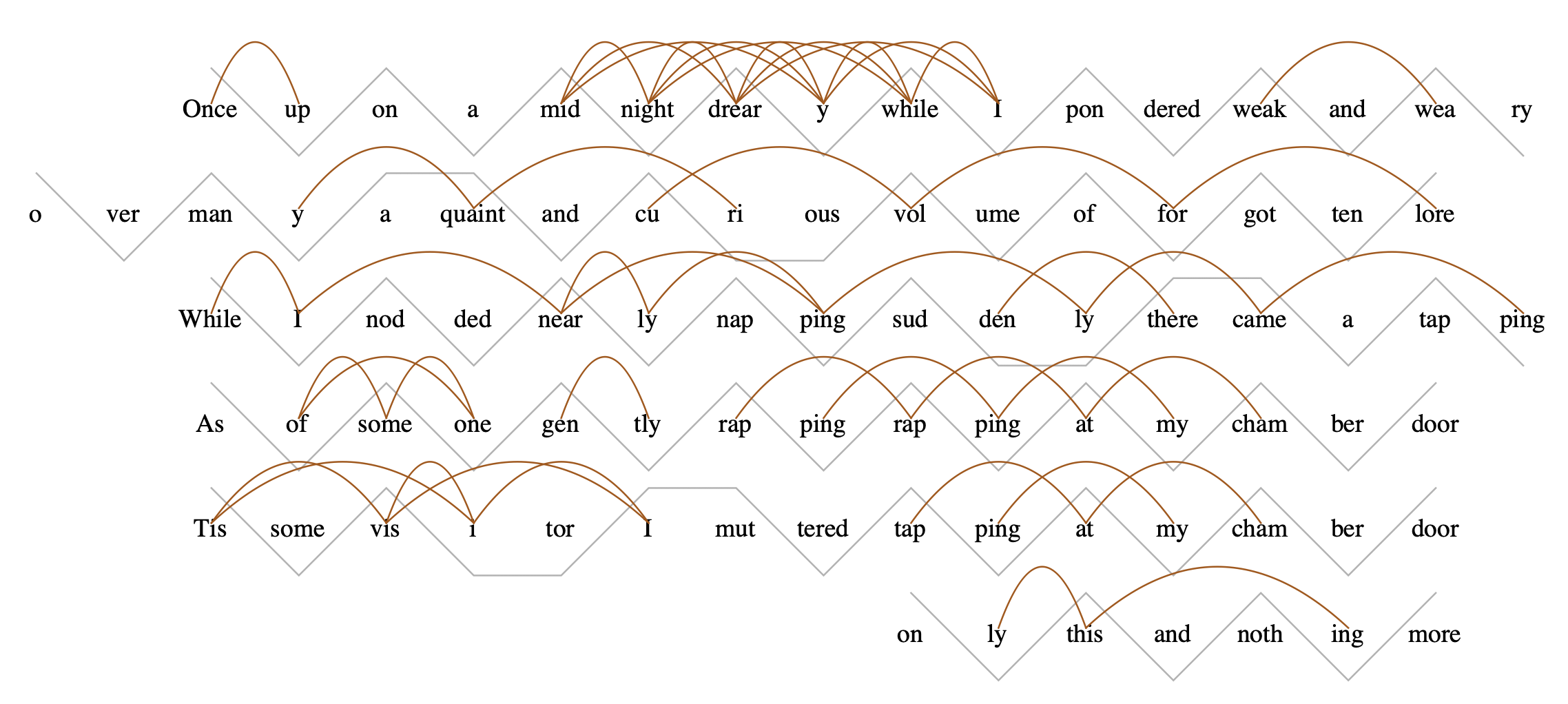
End Rhyme
The echoes of traditional end rhyme, what we commonly think of as rhyme, are more difficult to map because they can span multiple lines, they involve groups of syllables, and they are restricted to certain parts of the metrical pattern. This takes some prep work. I gather a list of rhyme candidates and then test them for phonetically identical endings. The results are somewhat better than with the other types of rhyme, and with some additional work I think I can eliminate most of the miscues. 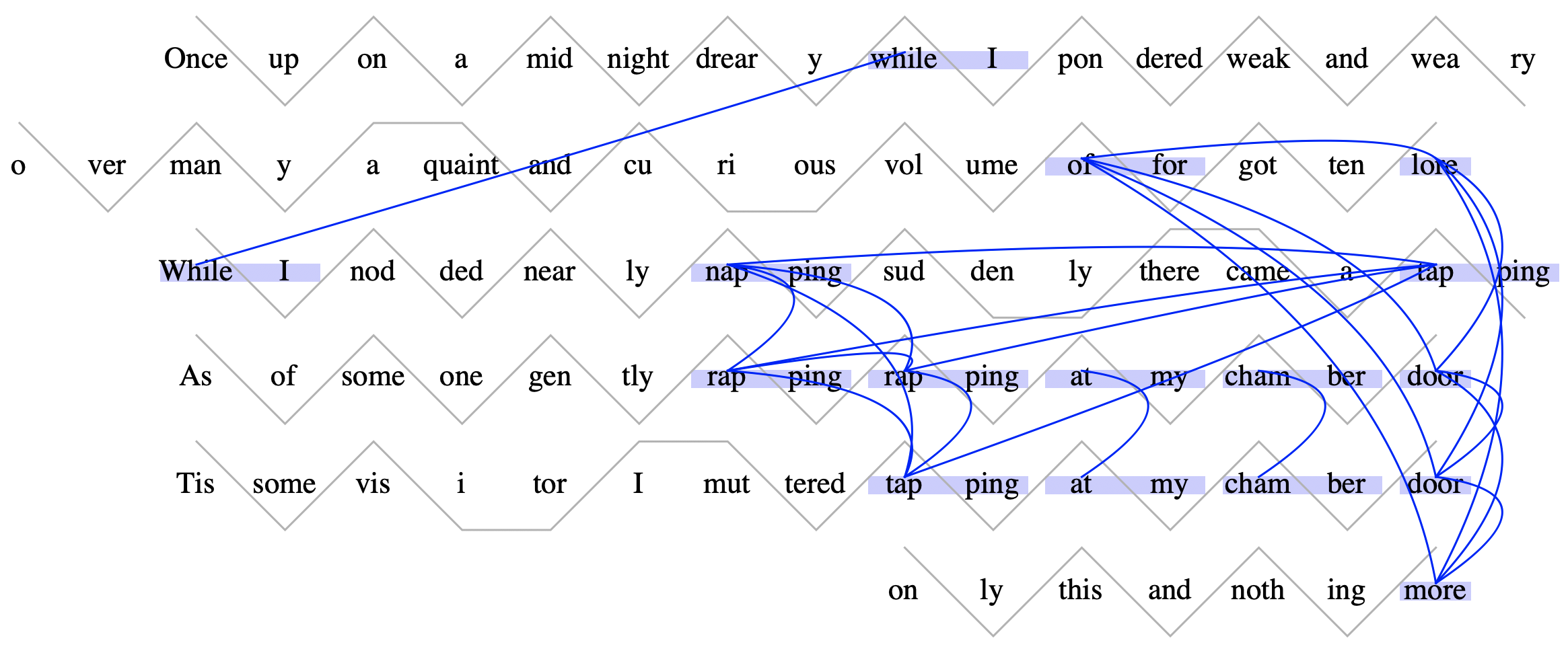
Conclusion
The point isnt so much that the computer catches every rhyme; perhaps in time it will. The point is that the computer can detect features in poetry and visualize them. Even with the mistakes this would be a good teaching tool. Questions like Can rapping rhyme with rapping? and Why does the computer think that while I is a candidate for rhyming? Are good discussion starters that lead to a deeper understanding of rhyme and the craft of poetry.
Adding machine learning to the analysis of meter in poetry and extending that to rhyme was my project at the Wolfram Summer School of 2019. Time was limited, so I didnt polish it as much as I would have liked. I do plan a Part 3 for this series on the cadence or pauses built into written poetry. I hope to also update the code for this section at that time.
Code
Below is the code for both Part 1 and Part 2, broken into sections with some comments interspersed.
Gathers training data for the new neural network that takes a word and returns the word's phonetic form and syllabification.
SeedRandom[1234];
allWords =
RandomSample[
Select[ToLowerCase[WordData[]],
StringMatchQ[LetterCharacter ..]]];
allWordsAssocs =
Quiet[DeleteMissing[<|"Input" -> #,
"FullTargetSequence" -> WordData[#, "PhoneticForm"],
"SyllabicTarget" -> WordData[#, "Hyphenation"]|> & /@ allWords,
1, 2]];
netInputData =
RandomSample[Fold[#2[#1] &, DeleteMissing[allWordsAssocs, 1, 2], {
MapAt[">" <> # <> "<" &, {All, "FullTargetSequence"}],
MapAt[
ToExpression[
Characters[
StringJoin[
StringReplace[#, {_ ~~ EndOfString -> "2", _ ->
"1"}]]]] &, {All, "SyllabicTarget"}]}]];
{train3, test3} =
TakeDrop[netInputData, Floor[Length[netInputData]*0.85]] ;
Column[RandomSample[test3, 4]]
Creates the encoders for the net.
ipaChars =
Sort@DeleteDuplicates@Flatten@Characters[netInputData[[All, 2]]];
inputEncoder = NetEncoder[{"Characters"}];
targetEncoder = NetEncoder[{"Characters", ipaChars}]
Creates the training configuration of the neural net.
net = NetGraph[
{
"charEnc" -> NetChain[{
EmbeddingLayer[32],
NetBidirectionalOperator[LongShortTermMemoryLayer[64]]
}],
"phoneticDec" -> NetGraph[
{
SequenceLastLayer[],
EmbeddingLayer[32],
GatedRecurrentLayer[128],
NetMapOperator[LinearLayer[]],
SoftmaxLayer[]
}
,
{
NetPort["State"] -> 1 -> NetPort[3, "State"],
NetPort["Input"] ->
2 -> 3 -> 4 -> 5 -> NetPort["phoneticOutput"]
}
],
"sylabicDec" -> NetGraph[
{
SequenceLastLayer[],
GatedRecurrentLayer[128],
NetMapOperator[LinearLayer[2]],
SoftmaxLayer[]
}
,
{
NetPort["Input"] -> 1 -> NetPort[2, "State"],
NetPort["Input"] -> 2 -> 3 -> 4 -> NetPort["sylabicOutput"]
}
]
}
,
{
"charEnc" -> NetPort["phoneticDec", "State"],
"charEnc" -> "sylabicDec",
NetPort["Target"] -> NetPort["phoneticDec", "Input"]
}
,
"Input" -> inputEncoder
]
Creates the loss mechanism for the net.
lossNet = NetGraph[
<|
"studentNet" -> net
, "sylabicLoss" -> CrossEntropyLossLayer["Index"]
, "phoneticLoss" -> CrossEntropyLossLayer["Index"]
, "toPredict" -> SequenceRestLayer[]
, "previousAnswer" -> SequenceMostLayer[]
, "finalLoss" -> ThreadingLayer[Plus]
|>
,
{
NetPort["FullTargetSequence"] ->
"toPredict" -> NetPort["phoneticLoss", "Target"],
NetPort["FullTargetSequence"] ->
"previousAnswer" -> NetPort["studentNet", "Target"],
NetPort["studentNet", "phoneticOutput"] ->
NetPort["phoneticLoss", "Input"],
NetPort["studentNet", "sylabicOutput"] ->
NetPort["sylabicLoss", "Input"],
NetPort["SylabicTarget"] -> NetPort["sylabicLoss", "Target"],
{"phoneticLoss", "sylabicLoss"} -> "finalLoss" -> NetPort["Loss"]
},
"FullTargetSequence" -> targetEncoder
]
Trains the net. I stopped at round 19 because the validation loss didn't improve after that.
netTrainRes =
NetTrain[lossNet, <|"Input" -> train3[[All, 1]],
"FullTargetSequence" -> train3[[All, 2]],
"SylabicTarget" -> train3[[All, 3]]|>, All,
ValidationSet -> <|"Input" -> test3[[All, 1]],
"FullTargetSequence" -> test3[[All, 2]],
"SylabicTarget" -> test3[[All, 3]]|>, MaxTrainingRounds -> 50]
Trims neural network to its production configuration.
trainedNet2 = netTrainRes["TrainedNet"];
phoTeachNet = NetDelete[trainedNet2, {"sylabicLoss", "finalLoss"}];
generationNet =
NetDelete[
trainedNet2, {"phoneticLoss", "sylabicLoss", "finalLoss",
"toPredict"}];
wordEncoding =
NetChain[{NetExtract[generationNet, {"studentNet", 1}]},
"Input" -> NetExtract[generationNet, "Input"]];
phoneticDec =
NetReplacePart[
NetDelete[NetExtract[generationNet, {"studentNet", "phoneticDec"}],
1], "Input" -> NetExtract[phoTeachNet, "FullTargetSequence"]];
phoneticDec =
NetReplacePart[
NetRename[
NetAppend[NetFlatten[phoneticDec],
SequenceLastLayer[]], {NetPort["Input"] ->
NetPort["PrevPhoneticChar"]}],
"Output" ->
NetDecoder[{"Class", targetEncoder[["Encoding"]]}]]; phoneticDec =
NetReplacePart[
NetDelete[NetExtract[generationNet, {"studentNet", "phoneticDec"}],
1], "Input" -> NetExtract[phoTeachNet, "FullTargetSequence"]];
phoneticDec =
NetReplacePart[
NetRename[
NetAppend[NetFlatten[phoneticDec],
SequenceLastLayer[]], {NetPort["Input"] ->
NetPort["PrevPhoneticChar"]}],
"Output" ->
NetDecoder[{"Class", targetEncoder[["Encoding"]]}]]; phoneticDec =
NetReplacePart[
NetDelete[NetExtract[generationNet, {"studentNet", "phoneticDec"}],
1], "Input" -> NetExtract[phoTeachNet, "FullTargetSequence"]];
phoneticDec =
NetReplacePart[
NetRename[
NetAppend[NetFlatten[phoneticDec],
SequenceLastLayer[]], {NetPort["Input"] ->
NetPort["PrevPhoneticChar"]}],
"Output" -> NetDecoder[{"Class", targetEncoder[["Encoding"]]}]];
syllabicDec =
NetExtract[generationNet, {"studentNet", "sylabicDec"}];
toStrChunks[x_Integer, y_] := {x, y};
toStrChunks[x_List, y_] := {Last[x] + 1, y};
Calls the neural net and displays some results.
getPhonsAndSylls[word_String] := Block[
{previousAnswer = ">", phonetic = "", syllabic},
Module[{wordenc = wordEncoding[word], stateNet},
(* decode the syllables *)
syllabic =
Flatten@Position[
syllabicDec[wordenc], {pNonBreack_, pBreack_} /;
pBreack > pNonBreack];
syllabic =
StringTake[word, Rest@FoldList[toStrChunks, 1, syllabic]];
(* decode the phonetic *)
stateNet =
NetStateObject[phoneticDec, <|{2, "State"} :> Last@wordenc|>];
While[previousAnswer =!= "<",
previousAnswer = stateNet[previousAnswer];
phonetic = phonetic <> previousAnswer];
<|"Phonetic" -> StringDrop[phonetic, -1],
"Syllables" -> syllabic|>]];
testSample = RandomSample[test3, 10][[All, 1]];
outputSample =
Flatten[{#, Values[getPhonsAndSylls[#]]}, 1] & /@ testSample;
sampleDataset =
Dataset[{<|"input" -> #[[1]], "phonetic" -> #[[2]],
"syllabic" -> #[[3]]|>} & /@ outputSample]
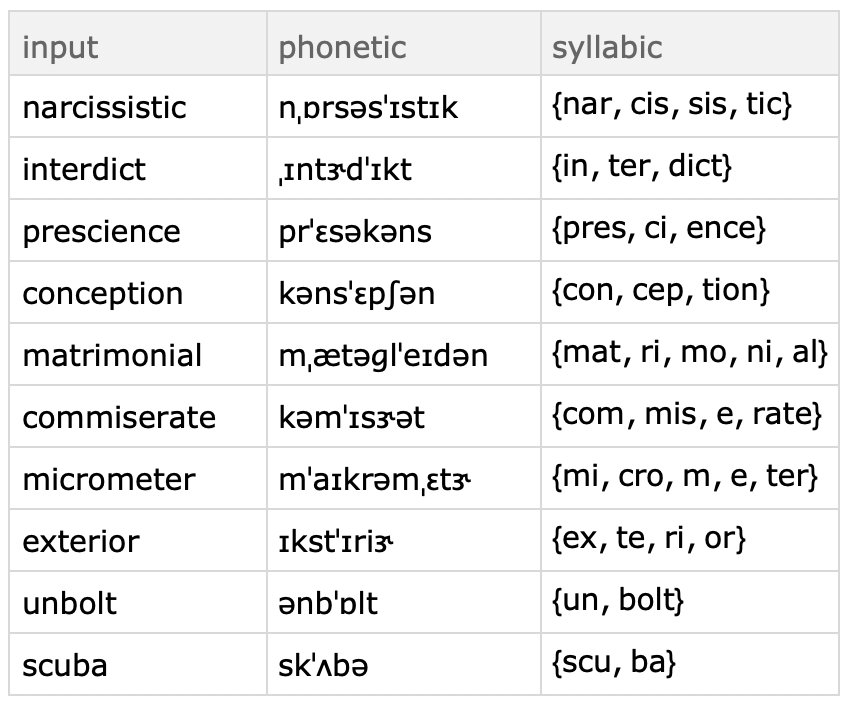
Gathers data for metric analysis.
ipaVowels = {"a?", "a?", "e?", "??", "o?", "?", "?", "?", "?", "?",
"?", "?", "?", "?", "?", "?", "?", "?", "?", "?", "?", "?", "?",
"?", "?", "a", "æ", "e", "i", "o", "", "ø", "u",
"y"}; oneSylStops =
Select[DeleteMissing[
WordData[#, "PhoneticForm"] & /@ WordData[All, "Stopwords"]],
StringCount[#, ipaVowels] < 2 &];
getPhoneticForm[word_] :=
Module[{phoneticForm},
WordData[ToLowerCase[word], "PhoneticForm"] /. _Missing ->
getPhonsAndSylls[word]["Phonetic"]];
getMeter[phon_] := Module[{ipaSylCt},
If[MemberQ[oneSylStops, phon], Return[{.5}]];
ipaSylCt = StringCount[phon, ipaVowels];
If[ipaSylCt == 1, {1},
vowelSounds = StringCases[phon, "?" | "?" ... ~~ ipaVowels];
# /. {a_ /; StringContainsQ[a, "?"] -> 1,
a_ /; StringContainsQ[a, "?"] -> .5, _ -> 0} & /@
vowelSounds]];
getSyllables[word_] := Module[{syllables, phonCheck, part1, part2},
phonCheck = StringCount[getPhoneticForm[word], ipaVowels];
If[phonCheck <= 1, Return[{word}]];
syllables =
WordData[ToLowerCase[word], "Hyphenation"] /. _Missing ->
getPhonsAndSylls[ToLowerCase[word]]["Syllables"];
If[Length[syllables] == 1 && phonCheck == 2,
vowels = {"a", "e", "i", "o", "u"};
part1 =
StringCases["over",
StartOfString ~~ Except[vowels] ... ~~ vowels ..][[1]];
part2 =
StringDelete["over",
StartOfString ~~ Except[vowels] ... ~~ vowels ..];
Return[{part1, part2}], Return[syllables]]];
getWordInfo[word_] := Module[
{phon = getPhoneticForm[ToLowerCase[word]]},
{word, getMeter[phon], getSyllables[word], phon}];
first10words = Take[TextWords[ToLowerCase[stanza]], 10];
first10info = getWordInfo[#] & /@ first10words;
first10assoc = <|"word" -> #[[1]], "meter" -> #[[2]],
"syllables" -> #[[3]], "phonetics" -> #[[4]]|> & /@ first10info //
Dataset
Displays metric graphic.
iambic = Table[Flatten[Table[{0, 1}, n]], {n, 1, 12}];
trochaic = RotateLeft[#] & /@ iambic;
anapestic = Table[Flatten[Table[{0, 0, 1}, n]], {n, 1, 12}];
dactylic = RotateRight[#] & /@ anapestic;
allPatterns = Join[iambic, trochaic, anapestic, dactylic];
analyzeMeter[stanza_] := (
lines = StringSplit[stanza, EndOfLine];
words = TextWords[#] & /@ lines;
wordInfo = Map[getWordInfo[#] &, words, {2}];
meter1 = Flatten[#] & /@ wordInfo[[All, All, 2]];
meter2 = meter1 //. {
{a___, 1, 1, 1, b___} -> {a, 1, 0, 1, b},
{a___, 1, 1, .5, b___} -> {a, 1, 1, 0, b},
{a___, .5, 1, 1, b___} -> {a, 0, 1, 1, b},
{a___, 0, 0, 0, b___} -> {a, 0, 1, 0, b},
{a___, 0, 0, .5, b___} -> {a, 0, 0, 1, b},
{a___, .5, 0, 0, b___} -> {a, 1, 0, 0, b}} ;
midCts = Count[#, .5, 2] & /@ meter2;
zero1s = Tuples[{0, 1}, #] & /@ midCts;
midPos = Flatten[Position[#, .5]] & /@ meter2;
prelim = MapIndexed[{midPos[[#2[[1]]]], #1} &, zero1s, {2}];
repRules = Map[(Rule @@@ Partition[Riffle @@ #, 2] &), prelim, {2}];
replaced =
MapIndexed[ReplacePart[meter2[[#2[[1]]]], #1] &, repRules, {2}];
seqPredict = SequencePredict[allPatterns];
result = seqPredict[#, "SequenceProbability"] & /@ replaced;
resultPos = Position[#, Max[#]][[1, 1]] & /@ result;
meter3 = MapIndexed[replaced[[#2, #1]][[1]] &, resultPos];
alignPt = Max[Position[#, 1]] & /@ meter3;
splitMeters = Partition[#, UpTo[Max[Position[#, 1]]]] & /@ meter3;
bkOff = Min[Position[Reverse[#], 1]] & /@ meter3;
coords = Flatten[MapIndexed[If[
#2[[2]] == 1,
{1 - #2[[3]], #1 + 1.2 (#2[[1]] - 1)},
{#2[[3]] - 1, #1 + 1.2 (#2[[1]] - 1)}
] &,
Reverse[MapAt[Prepend[1],
MapAt[Reverse,
splitMeters /. ({lhs_List} :> {lhs, {}}), {All, 1}], {All,
2}]], {3}], 1];
xRuns = Range[Length[#]] & /@ meter3;
lens = Length /@ meter3;
endPts = bkOff;
begPts = endPts - lens;
coordsX = MapThread[Range[#1, #2] &, {begPts + 1, endPts}];
coordsY = MapIndexed[{#1 + 1.2*#2[[1]]}[[1]] &, Reverse[meter3]];
coords2 =
MapThread[
Partition[Riffle[#1, #2], 2] &, {Reverse[coordsX], coordsY}];
txtCoo = MapIndexed[{#1[[1]], 1.2*#2[[1]] + .5} &, coords2, {2}];
syllab = #[[;; , 3]] & /@ Reverse[wordInfo];
textGr =
MapThread[
Style[Text[#1, #2], 15, FontFamily -> "Times"] &, {Flatten[
syllab], Partition[Flatten[txtCoo, 2], 2]}];
meterGraphic =
Graphics[{GrayLevel[.7], Line[coords2], Black, textGr},
ImageMargins -> {{10, 10}, {0, 0}}, ImageSize -> 900]
);
analyzeMeter[stanza]
Prepares information for rhyme graphics.
syllab2 = Flatten[#] & /@ Reverse[syllab];
phonSylls =
Flatten[MapIndexed[{StringDelete[
WordData[#1, "PhoneticForm"] /. _Missing ->
getPhonsAndSylls[#1]["Phonetic"], "?" | "?"], #2} &,
syllab2, {2}], 1];
allPairs = Subsets[phonSylls, {2}];
{Short[phonSylls], Short[allPairs]} // Column
Creates the alliteration graphic.
alliterationAll =
Cases[allPairs, {{ps1_, _}, {ps2_, _}} /;
Characters[StringDelete[ps1, "?" | "?"]][[1]] ==
Characters[StringDelete[ps2, "?" | "?"]][[1]]];
alliterationClose =
Cases[alliterationAll, {{_, {l1_, s1_}}, {_, {l2_, s2_}}} /;
l1 === l2 && Abs[s2 - s1] < 6];
alliterationCoords = {Reverse[txtCoo][[#[[1, 2, 1]], #[[1, 2, 2]]]],
Reverse[txtCoo][[#[[2, 2, 1]], #[[2, 2, 2]]]]} & /@
alliterationClose;
alliterationArcs =
BezierCurve[{#[[1]] + {0, .1},
Mean[{#[[1]], #[[2]]}] + {0, 1.5}, #[[2]] + {0, .1}}] & /@
alliterationCoords;
Show[meterGraphic, Graphics[{Darker[Green], alliterationArcs}]]
Creates the consonance graphic.
allPhonemes = ipaChars /. ("<" | ">" | "?" | "?") -> Nothing;
ipaConsonants = Complement[allPhonemes, ipaVowels];
consonanceAll =
Cases[allPairs, {{ps1_, _}, {ps2_, _}} /;
Length[Intersection[Characters[ps1], Characters[ps2],
ipaConsonants]] > 0];
consonanceClose =
Cases[consonanceAll, {{_, {l1_, s1_}}, {_, {l2_, s2_}}} /;
l1 === l2 && Abs[s2 - s1] < 4];
consonanceCoords = {Reverse[txtCoo][[#[[1, 2, 1]], #[[1, 2, 2]]]],
Reverse[txtCoo][[#[[2, 2, 1]], #[[2, 2, 2]]]]} & /@
consonanceClose;
consonanceArcs =
BezierCurve[{#[[1]] + {0, .1},
Mean[{#[[1]], #[[2]]}] + {0, 1.5}, #[[2]] + {0, .1}}] & /@
consonanceCoords;
Show[meterGraphic, Graphics[{Purple, consonanceArcs}]]
Creates the assonance graphic.
assonanceAll =
Cases[allPairs, {{ps1_, _}, {ps2_, _}} /;
Length[Intersection[Characters[ps1], Characters[ps2],
ipaVowels]] > 0];
assonanceClose =
Cases[assonanceAll, {{_, {l1_, s1_}}, {_, {l2_, s2_}}} /;
l1 === l2 && Abs[s2 - s1] < 4];
assonanceCoords = {Reverse[txtCoo][[#[[1, 2, 1]], #[[1, 2, 2]]]],
Reverse[txtCoo][[#[[2, 2, 1]], #[[2, 2, 2]]]]} & /@
assonanceClose;
assonanceArcs =
BezierCurve[{#[[1]] + {0, .1},
Mean[{#[[1]], #[[2]]}] + {0, 1.5}, #[[2]] + {0, .1}}] & /@
assonanceCoords;
Show[meterGraphic, Graphics[{Darker[Orange], assonanceArcs}]]
Creates the end rhyme graphic.
phonSyllsMeter =
MapThread[Append[#1, #2] &, {phonSylls, Flatten[meter3]}];
endRhymeMultiCans =
SequenceCases[
phonSyllsMeter, {{_, _, 1}, {_, _, 0},
Repeated[{_, _, 0}, {0, 1}]}];
endRhymeMultiSwords = {#[[1, 1]] <> #[[2, 1]], {#[[1, 2]], #[[-1,
2]]}} & /@ endRhymeMultiCans;
endRhymeUniCans =
Append[#[[1]] & /@
SequenceCases[
phonSyllsMeter, {{_, {a_, _}, 1}, {_, {b_, _}, _}} /; a != b],
phonSyllsMeter[[-1]]];
endRhymeUniSwords = {#[[1]], {#[[2]], #[[2]]}} & /@ endRhymeUniCans;
endRhymeSwords = Join[endRhymeMultiSwords, endRhymeUniSwords];
endRhymeSubsets = Subsets[endRhymeSwords, {2}];
endRhymes =
Cases[endRhymeSubsets, {{s1_, _}, {s2_, _}} /;
StringContainsQ[s1,
StringDelete[s2, StartOfString ~~ ipaConsonants ...]]];
endRhymeBgLt =
Rectangle[
Reverse[txtCoo][[#[[1, 2, 1, 1]], #[[1, 2, 1, 2]]]] + {-.4, -.1},
Reverse[txtCoo][[#[[1, 2, 2, 1]], #[[1, 2, 2,
2]]]] + {.4, .1}] & /@ endRhymes;
endRhymeBgRt =
Rectangle[
Reverse[txtCoo][[#[[2, 2, 1, 1]], #[[2, 2, 1, 2]]]] + {-.4, -.1},
Reverse[txtCoo][[#[[2, 2, 2, 1]], #[[2, 2, 2,
2]]]] + {.4, .1}] & /@ endRhymes;
endRhymeBg = Join[endRhymeBgLt, endRhymeBgRt];
endRhymeCoords = {Reverse[txtCoo][[#[[1, 2, 1, 1]], #[[1, 2, 1, 2]]]],
Reverse[txtCoo][[#[[2, 2, 1, 1]], #[[2, 2, 1, 2]]]]} & /@
endRhymes;
endRhymeArcs =
BezierCurve[{#[[1]] + {0, .1},
Mean[{#[[1]], #[[2]]}] + {1.5, .5}, #[[2]] + {0, .1}}] & /@
endRhymeCoords;
Show[Graphics[{RGBColor[.8, .8, 1], endRhymeBg}], meterGraphic,
Graphics[{Blue, endRhymeArcs}], ImageSize -> 800]