World's computation is gradually switching to GPUs and other SIMD devices, and if Mathematica doesn't adapt, it'll end up cornered as a tool for toy computations. Are there plans to make it easy to use GPUs in Mathematica?
You can see the reason for this in the graph below 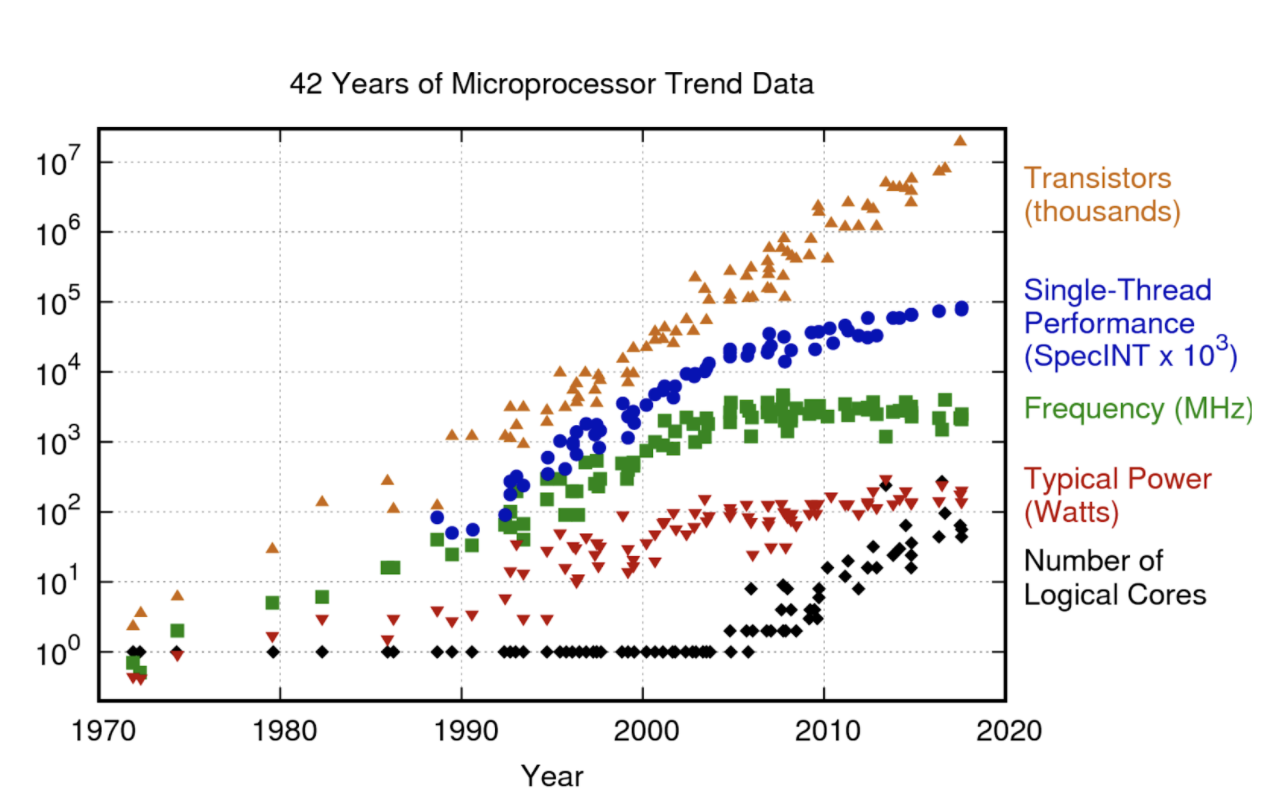
2006 witnessed the death of Dennard scaling and since them, much of new performance growth went into parallel computation rather than sequential. You can now buy a gaming card RTX 3090 with 36 teraflops of computation, compared to something like 200 gigaflops for CPU, and the gap is growing. These SIMD flops have gotten so cheap, that companies like Google are giving them out for free (https://colab.research.google.com/ runs things for free on a low-end GPU)
As a concrete example is this issue. I couldn't find an easy way to move this computation to GPU so had to reimplement it in Python. The changes you need to switch from CPU to GPU are minimal in modern numpy-like frameworks (ie, Jax, CuPY, PyTorch): add ".cuda()" in front of some tensors and their computations are automatically on GPU.
Mathematica could use similar approach to gradually enter the realm of user-friendly GPU computation -- first add an operation to move an array to GPU and back, and then implement GPU version of operation for the most common operations (matrix multiply), then gradually expand to more operations requested by users.