Hey, thanks for the award! But perhaps we're moving too fast? The previous code doesn't do depth-first searching, and we haven't even solve the eight queens puzzle yet! Let's keep going on this one...
There is a BacktrackSearch available through WFR, but it seems to have a design flaw. Summer school students: Anyone can tell what is the flaw? If not, try comparing with this modified version of the source code, not too different really:
BacktrackSearch[init_, levelData_, stateUpdate_,
solutionQ_, max : (_Integer?Positive | All) : 1] := Module[{
state = {init}, nextState, depth = Length[levelData], res = {},
index, level = 1, solution, count = max},
index = ConstantArray[0, {depth}];
While[level > 0,
nextState = Nothing;
While[SameQ[nextState, Nothing] && (
index[[level]] < Length[levelData[[level]]]),
index[[level]]++;
nextState = stateUpdate[Last[state],
levelData[[level, index[[level]]]] ];];
If[SameQ[nextState, Nothing],
state = state[[1 ;; -2]]; index[[level--]] = 0,
AppendTo[state, nextState]; level++];
If[level > depth,
If[solutionQ[Last[state]],
If[max === 1,
res = state;
level = 0,
AppendTo[res, state];
If[--count == 0, level = 0];
];];
state = state[[1 ;; -2]]; level--]
]; res]
Except that it now uses a stateUpdate function to compute partial solutions along the way. How does such an innovation improve timing statistics?
And for sake of reference, here's another similar Backtrack search function (of our own design):
DepthFirstIterate[stateUpdate_, solutionQ_,
levelData_, levelLens_, maxLevel_, count_
][state_, inds_, level_] := Module[
{nextState, res = Nothing, allRes = {},
ind = 1, len = levelLens[[level]]},
If[level == maxLevel + 1,
If[solutionQ[Last[state]],
Return[{state}], Return[{Nothing}]]];
While[ind <= len,
nextState = stateUpdate[Last[state], levelData[[level, ind]] ];
If[SameQ[Nothing, nextState], ind++,
res = DepthFirstIterate[stateUpdate, solutionQ,
levelData, levelLens, maxLevel, count - Length[allRes]][
Append[state, nextState], Append[inds, ind], level + 1];
allRes = Join[allRes, res];
If[Length[allRes] == count, Break[]];
ind++]]; allRes]
DepthFirstSearch[init_, levelData_, stateUpdate_,
solutionQ_, count : (_Integer?Positive | All) : 1] := Block[
{$RecursionLimit = Infinity}, With[{
levelLens = Append[Length /@ levelData, 0],
depth = Length@levelData},
If[SameQ[Length[#], count, 1],
First[#], #] &[
DepthFirstIterate[
stateUpdate, solutionQ,
levelData, levelLens, depth, count
][{init}, {}, 1]]]]
Now we actually have not one, but three possible methods for GenerateTiling. For the sake of clarity we will put the new methods into another function GenerateCover and wrap that with GenerateTemplatePattern:
PadTemplate[template_, size_] := With[
{colLen = size[[2]] + Dimensions[template][[2]] - 1},
Join[PadRight[#, colLen, _] & /@ template,
Table[ConstantArray[_, colLen], {size[[1]] - 1}]]]
AllTemplates[template_, size_] := With[{
padded = PadTemplate[template, size]},
Catenate@Outer[Function[{row, col},
RotateRight[RotateRight[#, col] & /@ padded, row
][[-size[[1]] ;; -1, -size[[2]] ;; -1]]],
Range[0, size[[1]] + Dimensions[template][[1]] - 2],
Range[0, size[[2]] + Dimensions[template][[2]] - 2],
1]]
ElementsCombine[Verbatim[_], x_] := x
ElementsCombine[x_, Verbatim[_]] := x
ElementsCombine[x_, y_] := If[SameQ[x, y], x, Throw[Nothing]]
RowsCombine[row1_, row2_] :=
Catch[MapThread[ElementsCombine, {row1, row2}]]
ReadByDiagonals[size_] :=
With[{ord = Catenate@Table[{i, j}, {i, size[[1]] }, {j, size[[2]] }]},
Position[ord, #][[1, 1]] & /@ SortBy[ord, {Total[#], #} &]]
GenerateCover[method : (DepthFirstSearch | BacktrackSearch),
levelData_, stateUpdate_, size_List, 1] :=
If[SameQ[#, {}], $Failed,
Partition[Last[#], size[[2]]]
] &@method[Table[_, Times @@ size],
levelData, stateUpdate, True &, 1]
GenerateCover[method : (DepthFirstSearch | BacktrackSearch),
levelData_, stateUpdate_, size_List, count_] :=
If[SameQ[#, Nothing], $Failed,
Partition[Last[#], size[[2]]]] & /@ method[Table[_, Times @@ size],
levelData, stateUpdate, True &, count]
GenerateCover["BreadthFirst", levelData_, stateUpdate_, size_List,
count_ : All (*useless here*)] :=
Map[Partition[#, size[[2]]] &, Fold[
Union@Catenate[Outer[stateUpdate, #1, #2, 1]] &,
{Table[_, Times @@ size]}, levelData]]
GenerateCover[levelData_, stateUpdate_, size_List,
opts : OptionsPattern[{Method -> "BreadthFirst", Count -> 1}]
] := GenerateCover[OptionValue[Method],
levelData, stateUpdate, size, OptionValue[Count]]
GenerateCover[levelData_, stateUpdate_, size_Integer,
opts : OptionsPattern[{Method -> "BreadthFirst", Count -> 1}]
] := GenerateCover[levelData, stateUpdate, {size, size}, opts]
TemplatesToLevelData[templates_, size_Integer
] := TemplatesToLevelData[templates, {size, size}]
TemplatesToLevelData[templates_, size_List] := Transpose[
(Catenate /@ AllTemplates[#, size]) & /@ templates
][[ReadByDiagonals[
size + Dimensions[templates][[2 ;; 3]] - {1, 1}]]]
GenerateTemplatePattern[templates_, size_,
opts : OptionsPattern[{Method -> "BreadthFirst", Count -> 1}]
] := GenerateCover[TemplatesToLevelData[templates, size],
RowsCombine, size, opts]
Now let's see what it can do. For a shallow search on an easy tile set, depth first goes about as fast as breadth first:
tempTest = Union@Flatten[Partition[Table[Mod[i + j, 4],
{i, 0, 6}, {j, 0, 6}], {2, 2}, {1, 1}], 1];
AbsoluteTiming[
Length@GenerateTemplatePattern[tempTest,
20, Method -> #, Count -> All]
] & /@ {"BreadthFirst", DepthFirstSearch, BacktrackSearch}
Out[] = 1.4, 1.4, 1.4
If we just want to generate one template-consistent pattern, we can pass Count option to one of the backtrackers:
ArrayPlot[GenerateTemplatePattern[tempTest,
20, Method -> DepthFirstSearch, Count -> 1],
ColorRules -> {1 -> Red, 2 -> Green, 3 -> Blue, 0 -> Yellow}]
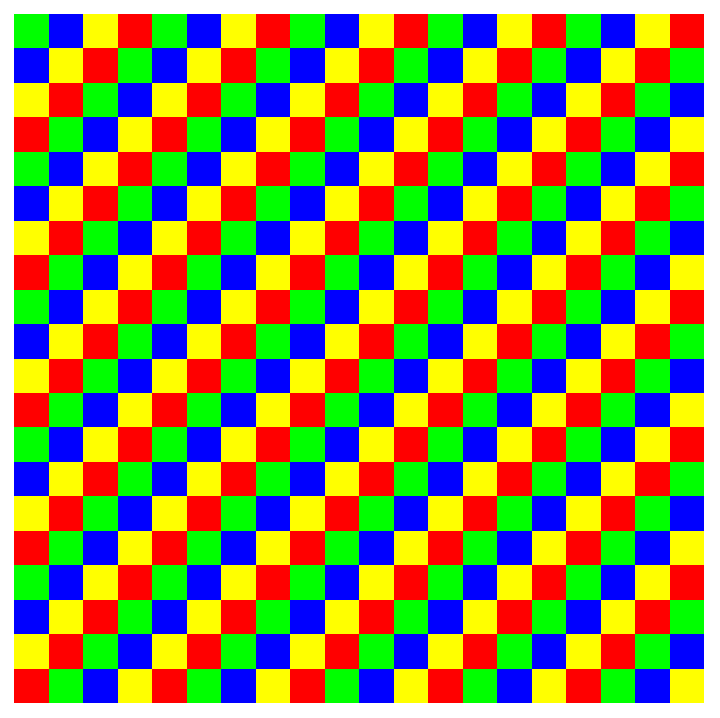
And the relevant times are:
AbsoluteTiming[
Length@GenerateTemplatePattern[tempTest,
20, Method -> #, Count -> 1]
] & /@ {"BreadthFirst", DepthFirstSearch, BacktrackSearch}
Out[] = 1.3, 0.35, 0.35
The times for DepthFirstSearch and BacktrackSearch indicate the algorithms are doing the same things in the same times, even though they are written slightly different. Both methods are much faster than what we currently have at WFR, which takes an unreasonable 400 seconds to do a similar calculation.
That's a much bigger slowness factor than we get comparing to GenerateTiling :
GenerateTiling = ResourceFunction["GenerateTiling"];
AbsoluteTiming[GenerateTiling[tempTest, {}, 20];][[1]]
Out[] = 0.1
Now we can generate some data by varying the pattern size, and plot:
dataGT1 = AbsoluteTiming[Length@GenerateTiling[tempTest, {}, #]] & /@ Range[20];
dataGC1 = AbsoluteTiming[Length@GenerateTemplatePattern[tempTest,
#, Method -> BacktrackSearch, Count -> 1]] & /@ Range[20];
Row[Show[#, ImageSize -> 250] & /@ {
ListPlot[{Reverse /@ dataGT1, Reverse /@ dataGC1},
PlotRange -> All],
Show[Plot[1, {x, 0, 20}],
ListPlot[dataGT1[[All, 1]]/dataGC1[[All, 1]]],
PlotRange -> All]}, Spacer[20]]
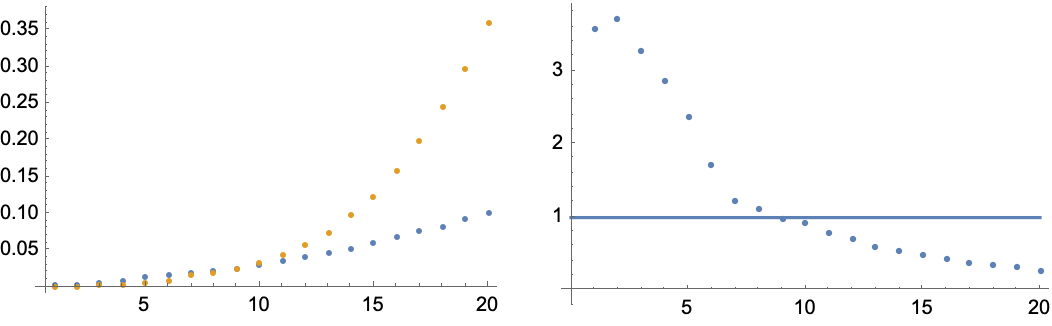
This data actually recapitulates what other researchers have found, that SAT solving methods can lose on the low end to backtracking algorithms, while still going faster in the long run. Here the crossover occurs for a grid size about
$10 \times 10$.
Perhaps we could potentially save time in comprehensive subset searching doing small-grid eliminations using the cover method? Yes, it's a possibility, but only if breadth first is fast enough. As inputs become more complex, search trees get deeper and backtracking algorithms can easily get lost in state space and end up wasting time. Perhaps beam searching could be useful, but often the answer for getting better times is not to make things more complicated.
Instead of worrying more about times, let's talk about scope. Backtracking algorithms are a decent way to solve a wide range of combinatorial problems (some of which could even be quite practical). Some of the typical examples are solving sudoku or the eight queens puzzle:
With[{data = DeleteDuplicatesBy[
GenerateCover[Map[Flatten,
QueenCoversData[{8, 8}], {2}],
RowsCombine, {8, 8}, Method -> "BreadthFirst"],
Sort[ResourceFunction["ArrayRotations"][#]] &] /. {1 -> 2}},
Grid[Partition[
ResourceFunction["CharacterArrayPlot"][
Plus[#, Table[Mod[i + j, 2], {i, 8}, {j, 8}]],
ColorRules -> {x_ :>
Association[0 -> Lighter[Yellow, .6],
1 -> Lighter[Orange, .6]][Mod[x, 2]]},
"CharacterRules" -> {1 -> "", 0 -> "",
x_ :> FromCharacterCode[9819]},
"CharacterStyleRules" -> {x_ :> {16, Black}},
MeshStyle -> Directive[Gray, Thick],
ImageSize -> 200
] & /@ data, 3], Spacings -> {1, 1}]]
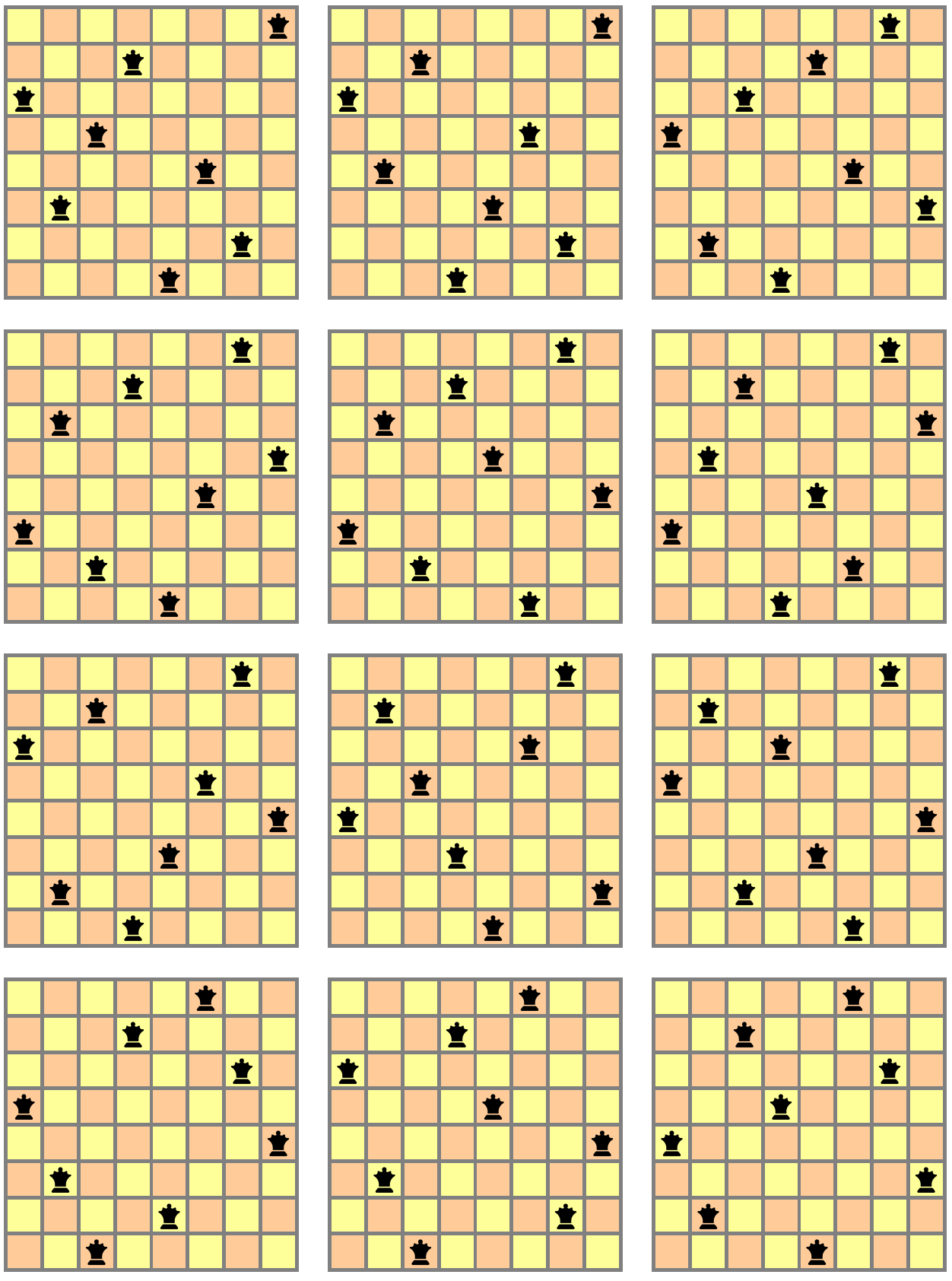
It's really an easy problem. To calculate the entire set of 92 it took me only 0.4 seconds, and to calculate just one solution, only .05 seconds. But I left out the code, how did I do this? Can any of the summer school students do much better?