This one shows the first rule that applies to the first active cell, the second rule that applies to second active cell, and so on. Given a collection of cells on a state for which the system is given rules that can generate multiple outputs which can be expected to generate more possibilities of connectivity, one cell can be updated at each step.
With[{g = MultiwayMobileAutomaton[{{275, 999}, {81, 384}},
{{ConstantArray[0, 35], 17}}, 5, "StatesGraphStructure",
VertexSize -> 0.5]},
HighlightGraph[g, {VertexOutComponent[g, VertexList[g][[1]], 2]}]]
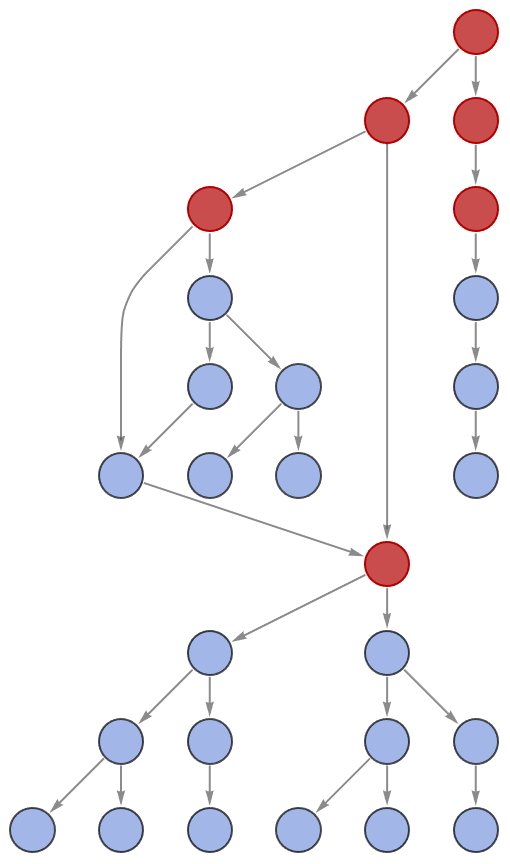
You can combine rules for more connectivity in the multiway systems, like how 4 rules are combined & update the states 6 times or how you're getting all these rules within a specific combination of cells. Did you know that branchial graphs are accurate, ahistorical or as the rules apply in one step?
g = MultiwayMobileAutomaton[{{275, 999}, {81,
384}}, {{ConstantArray[0, 11], 5}}, 3, "StatesGraph",
VertexSize -> 1]
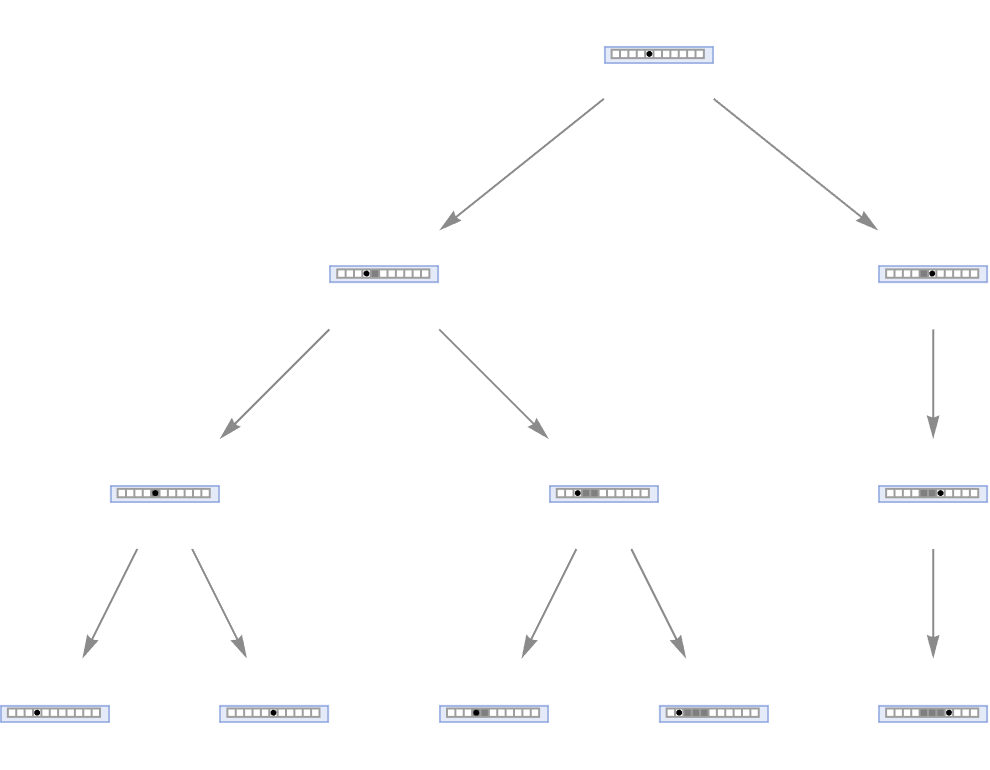
Running the systems for a few more steps, one can start to see more complex stuff at each step when we see more branching and merging and just want to know what part of the orbit it's going to be. Representing the position of the active cells after an update (grey or white), mapping those three blocks of state via rules that will be specified as a list of two numbers to where the updated cell will turn after an update, and also as a binary list.
g = MultiwayMobileAutomaton[{{275, 999}, {81,
384}}, {{ConstantArray[0, 34], 16}}, 15, "StatesGraphStructure",
VertexSize -> 0.5]
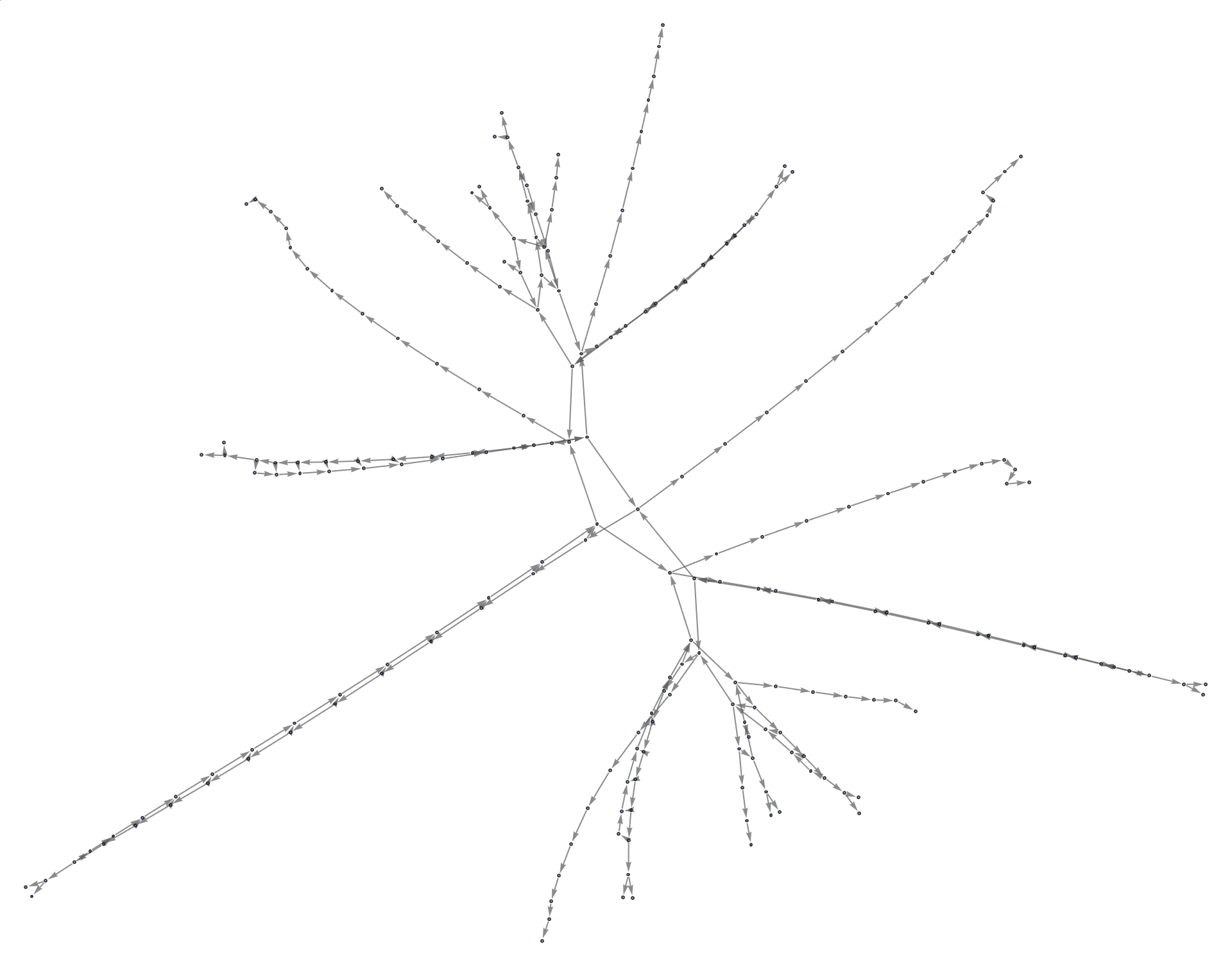
Ordinarily, apply the same rules to the same configuration of the state being updated, which generates many possible paths of evolution. I didn't know that only one active cell could get updated at a time according to a specific rule! That's what it's like going from ordinary to multiway. It's the intermediate case between the combined rules of single-headed Mobile Automata and multi-way string substitution systems. It's like combining multiple rules of ordinary Turing Machines to create a multi-way Turing Machine.
With[{start = ToString@{ConstantArray[0, 35], 17}},
Table[
Graph3D[
HighlightGraph[
MultiwayMobileAutomaton[rule, {start}, 7, structure,
VertexSize -> 1.2], start]],
{rule, {{{700, 100}, {761, 851}}, {{295, 265}, {218, 912}}, {{21,
78}, {550, 788}}, {{312, 238}, {707, 502}}, {{272, 239}, {283,
592}} }}, {structure, {"BranchialGraphStructure",
"StatesGraphStructure"}}
]]
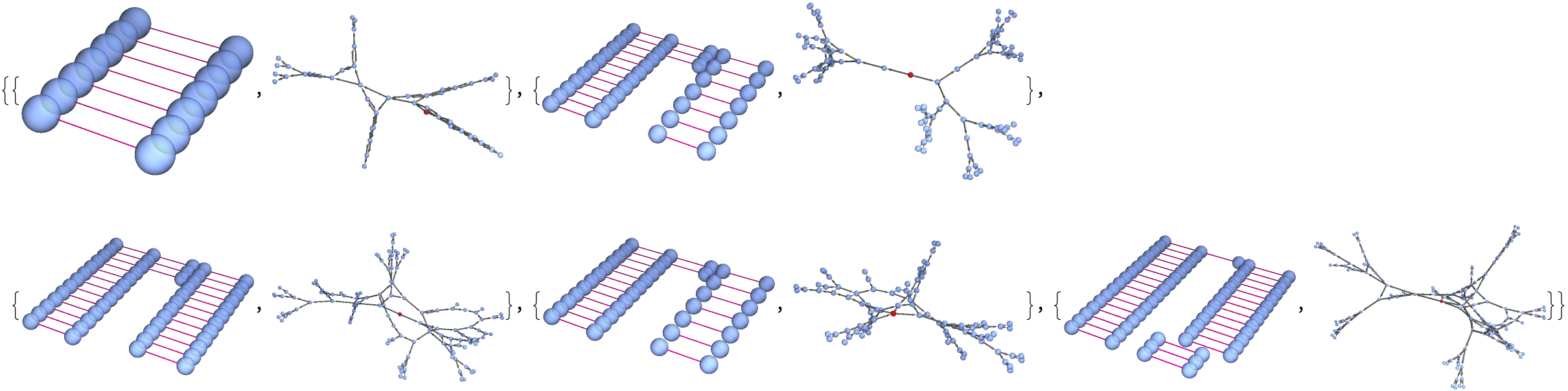
I really like this post, that was some retrospective how you did it with a list of two numbers and got the color and position.
With[{
g = MultiwayMultiHeadedAutomaton[
{{275, 999}, {81, 384}},
{{ConstantArray[0, 35], {17, 18}}},
5,
"StatesGraphStructure",
VertexSize -> 0.5]},
HighlightGraph[g, {
VertexOutComponent[g,
VertexList[g][[1]],
2]}]]
evolution = {
{{0, 0, 0, 1, 0, 0, 0}, {3}},
{{0, 0, 1, 1, 0, 0, 0}, {2, 4}},
{{0, 1, 1, 1, 0, 0, 0}, {2, 3, 4}}
};
MobileAutomatonPlot[evolution, Mesh -> True]
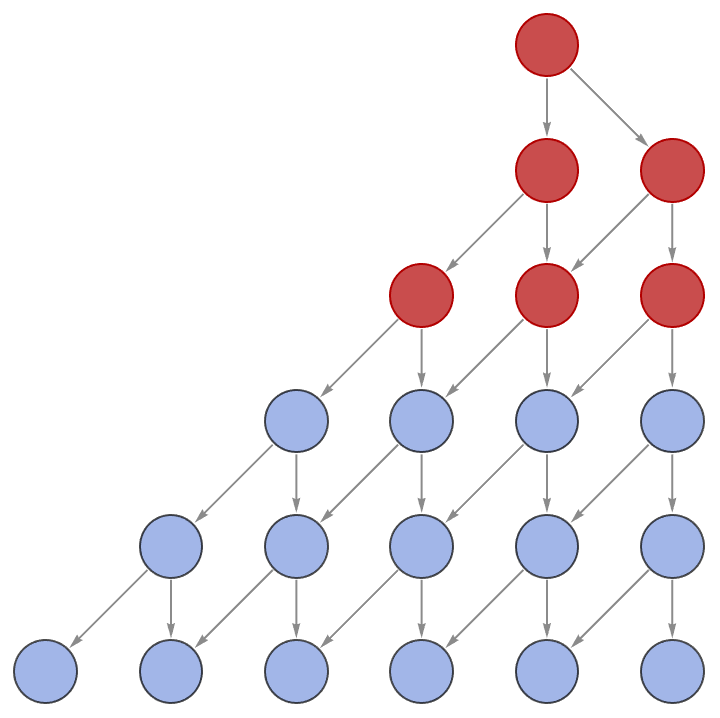
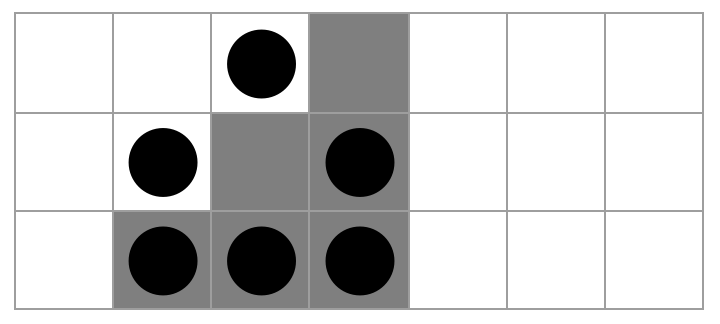
Names. @Felipe Amorim with all these MultiwayMultiHeadedAutomaton
what we're really doing is with multiple "heads" that are really separate processing units..if you wanted you could evolve the automaton for 5 steps and position yourself..highlight it, highlight it. Now, the rule of the automaton in the form of outcomes and their patterns, highlights the cell divisions.
Manipulate[
evolution =
CellularAutomaton[{rule, {3, 1}}, {initialState, 0}, step];
ArrayPlot[
evolution,
ColorFunction -> ColorData[colorData],
ColorFunctionScaling -> False,
Frame -> False,
Mesh -> mesh,
MeshStyle -> Directive[GrayLevel[0.9], Dashed],
LabelStyle -> Directive[Bold, Larger],
AspectRatio -> 0.5,
ImageSize -> 600,
PlotRangePadding -> 0,
PlotLegends ->
BarLegend[{ColorData[colorData], {0, 1}}, LegendFunction -> "Panel"]
],
{{rule, 30, "Rule"}, 0, 255, 1, Appearance -> "Labeled"},
{{step, 50, "Step"}, 0, 200, 1, Appearance -> "Labeled"},
{{initialState, {0, 0, 0, 0, 1, 0, 0, 0, 0}, "Initial State"},
ControlType -> InputField},
{{colorData, "SunsetColors", "Color Data"}, ColorData["Gradients"],
ControlType -> PopupMenu},
{mesh, {True, False}, ControlType -> Checkbox}]
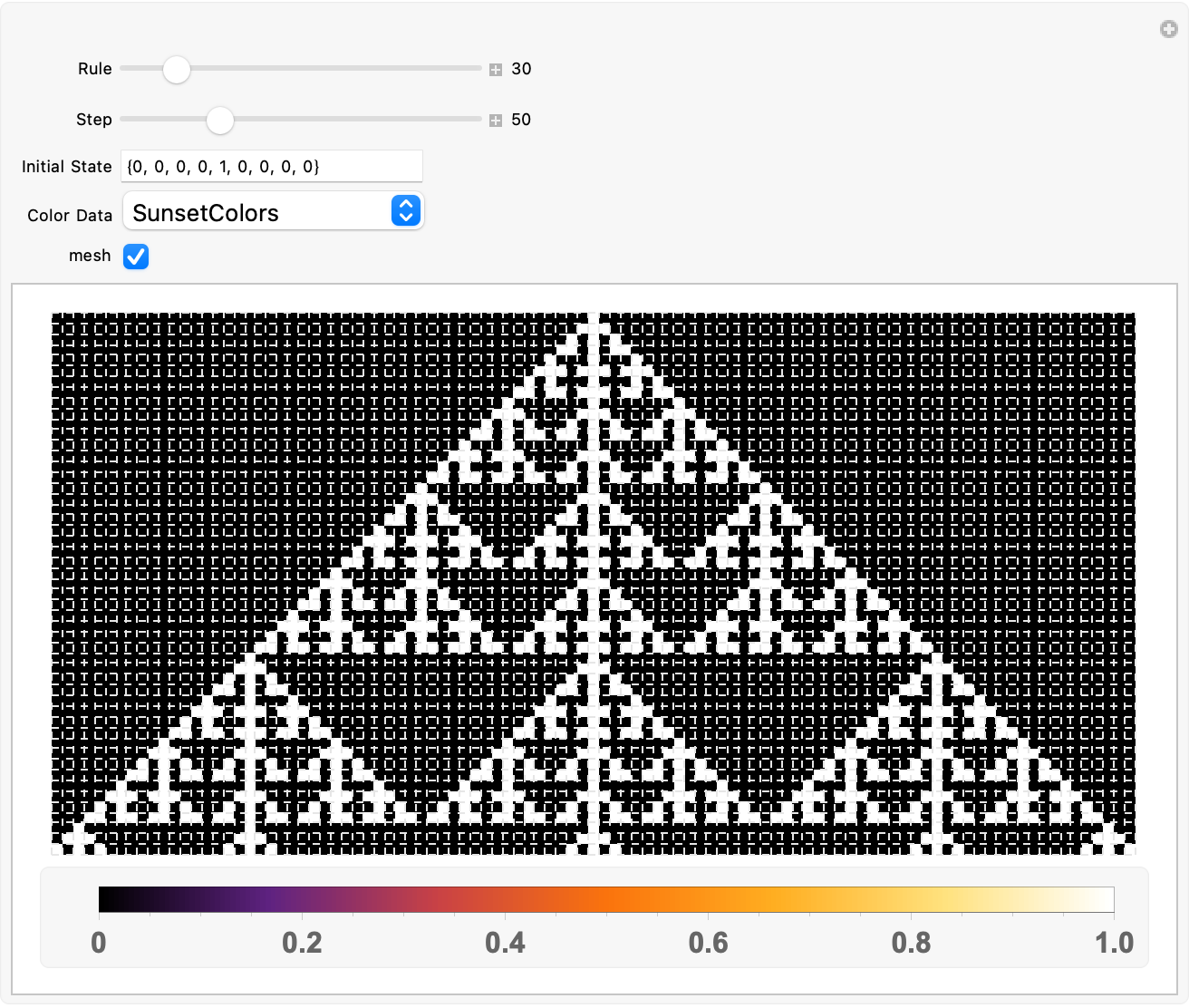
These cellular automata, it takes a while..now that you've got the Mathematica visualization of the 3-color cellular automata on one dimension within which the visualization of the ArrayPlot
and the implementation of the controls for these values..0, 1, 2, ...first when you turn the geological clock, and the code hidden within the language.
Table[
MultiwayMultiHeadedAutomaton[
{{275, 999}, {81, 384}},
{{ConstantArray[0, 34], {16, 16}}},
step,
"StatesGraphStructure",
VertexSize -> 0.5
],
{step, 1, 15}
]
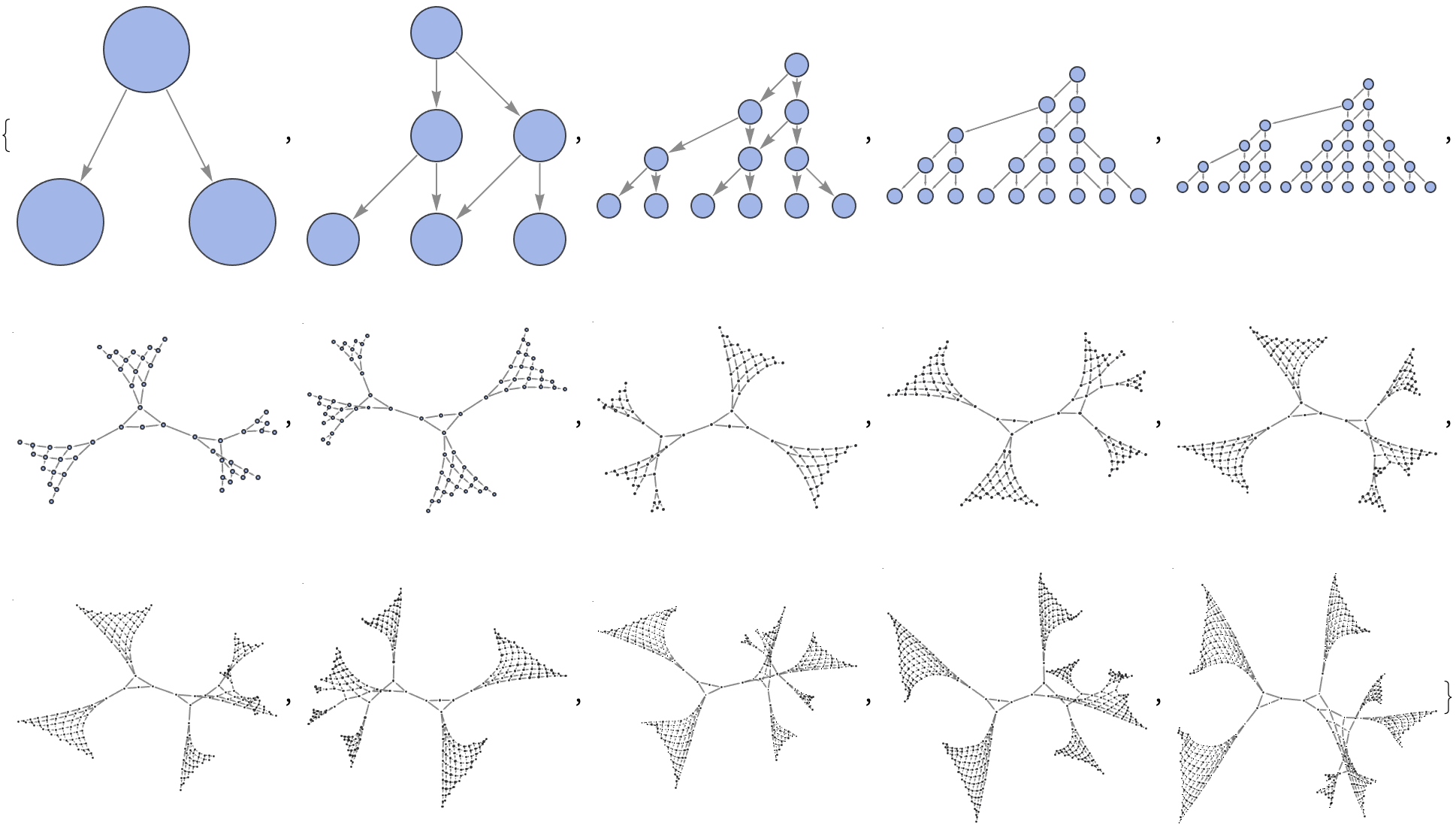
I care about the "multi-headed" cellular automaton, not the graph of states produced. You know how it goes, it's really about the two rules {275, 999}
and {81, 384}
that create the evolution that serves as the foundation for the sequence of evolution steps. That and the binary list of 34 zeros with two heads, both at the 16th index. I wonder if that could be edited.
statesNew[rule_, state_List] := If[
ListQ[state[[1]]] && Length@state[[1]] > 0,
CellularAutomaton[rule, {Flatten[state], 0}, {{0}}],
state]
getMAStateGraphics[rule_, state_] := ArrayPlot[state,
Mesh -> True,
ColorRules -> {0 -> White}]
stateRenderingFunction[rule_] :=
Inset[getMAStateGraphics[rule, ToExpression[#2]], #1, Center, #3] &
statesEvolutionFunction[rules_List, initial_List] := Map[
With[{new = statesNew[rules[[#]], initial[[#]]]},
{new, ReplacePart[initial, # -> new]}] &,
Range@Length[rules]]
MultiwayMultiHeadedAutomaton[rules_List, init_List, nSteps_Integer,
rest___] := ResourceFunction["MultiwaySystem"][
Association[
"StateEvolutionFunction" -> (statesEvolutionFunction[rules, #] &),
"StateEquivalenceFunction" -> SameQ,
"StateEventFunction" -> (# &),
"EventDecompositionFunction" -> Function[{a, b, c}, None],
"EventApplicationFunction" -> Function[{a, b, c}, None],
"SystemType" -> "MobileAutomaton",
"EventSelectionFunction" -> Identity],
init, nSteps, rest,
"StateRenderingFunction" -> stateRenderingFunction[First[rules]],
"EventRenderingFunction" -> Function[{a, b, c}, None]]
With[
{
g = MultiwayMultiHeadedAutomaton[
{{30, 2}, {90, 2}},
{{0, 0, 0, 1, 0, 0, 0, 0, 0}, {0, 0, 1, 1, 0, 0, 0, 0}},
10,
"StatesGraphStructure",
VertexSize -> 5]
},
HighlightGraph[g,
{VertexOutComponent[g, VertexList[g][[1]], 2]}
]]
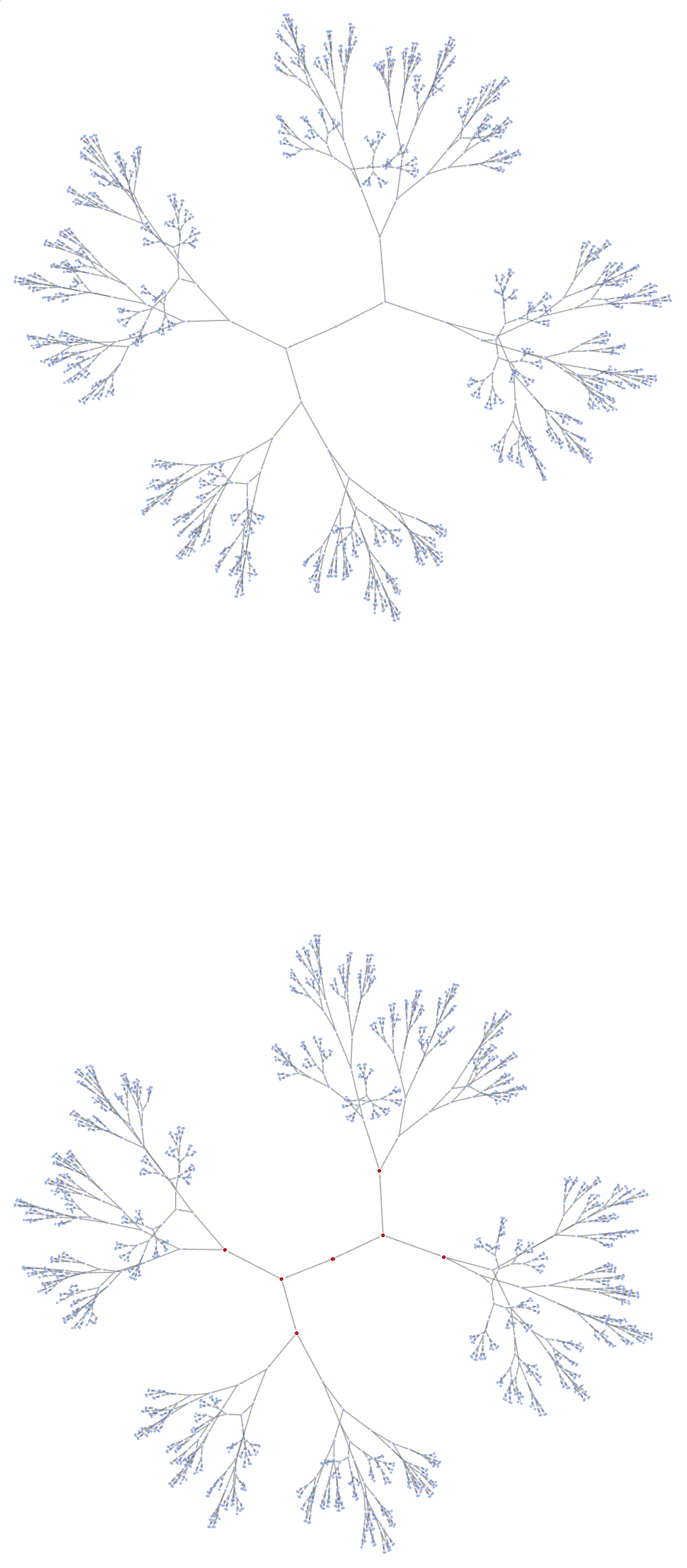
Now that you define and execute the multi-headed cellular automaton with even more than one active cell in a single step, how can it be that you can even create a graphical representation of a state? It looks so good. Your multiway mobile automata looks so good in this one.
The MultiwayMultiHeadedAutomaton
function...relies on the rules & states being used...uses MultiwaySystem
to define the new system behavior!