Hello everybody,
First of all let me say that i am glad to be here. This will be my very first post. I am a student at Virginia Tech, and my major is Industrial Engineering. The topic that I have some questions about are not discussed in my curriculum; however, I have become very interested in the past 6 months. Mathematica has been great in helping me visualize and understand some of the concepts, even-though i am as new to this program as I am to the subject. I will try to make my question as clear as possible.
If I define a simple process as such:
simpleproc[[Mu]_, [Sigma]_] = ItoProcess[
[DifferentialD]x[t] == [Mu]*[DifferentialD]t + [Sigma] [DifferentialD]w[t], x[t], {x, 0}, t, w [Distributed] WienerProcess[]];
Since, mu and sigma are constant - I can take the observed data for variable " x " - estimate the parameters for a Normal Distribution then directly substitute those values to the drift, and volatility of the diffusion. Easy so far.
Now I am attempting to model a Stochastic Damped Harmonic Oscillator with a random driven force, which I found in a book called "Modeling with Ito Stochastic Differential Equations" from Dr. Allen.E. Here is the model:
Eq 1. d x(t) = v(t) dt Eq. 2 d M(t) = (-k x(t) - b v(t)) dt + Sqrt(2 gamma^2 lamda) d W(t),
where M is the momentum, k is the coefficient of friction, b is the damping coefficient, and most importantly gamma and lambda are change in momentum, and the unknown probability of that change for a dt, respectively.
Since, the above equations have x, v and m - I divided both sides of equation 2 by 100 kg (fixed mass) since d M = m * d v. The we get:
proc[k_, b_, [Lambda]_, [Gamma]_] = ItoProcess[{
[DifferentialD]x[t] == v[t] [DifferentialD]t,
[DifferentialD]v[t] == -((b/100) v[t] + (k/100) x[t]) [DifferentialD]t + ((Sqrt[2 [Lambda] [Gamma]^2])/100) [DifferentialD]n[t]},
x[t], {{x, v}, {1, 0}}, t, n [Distributed] WienerProcess[]]
so, given a time series of observed accelerations in one direction - which I measured with my phone... How do fit such data to this type of SDE?
What I find hard to understand is that the the drift of this diffusion is the driving equation: hence, there is nothing there to fit. If I assume that changes in acceleration are Normally Distributed (which they are) with mean 0, and say sd = alpha. I still have two variables in the volatility (gamma, lambda);
...and lambda is a probability.
The only thing that I can think of to do (which may be incorrect) is to let lambda be "RandomReal," and gamma which now is the change in velocity equal to the expectation of the acceleration computed from the observed data. Then, I can simulate as many paths and take time slices or whatever.
However, simulation does not allow me to parametrize the process from data like I could with the simple first example, and that is not good.
I can clarify further if needed.
Thank you in advance! Any help would be greatly appreciated, and take it easy on me - I am new at this.
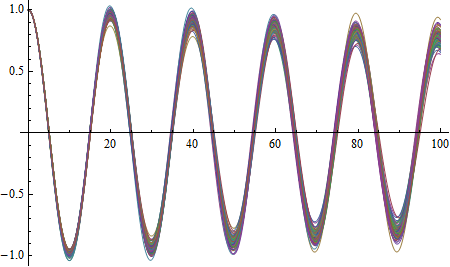