Dear Community! Although gambling is not my hobby, the validation of predictor engine inside Wolfram Mathematica 11.2 is. Sooner or later someone may get huge benefits in gambling using predictors as they have been developed already today. I got LaLiga "Outcomes" 100 % but my example will be Italian Serie A analysis, yrs 1934-2017. It is downloadable from
https://github.com/jalapic/engsoccerdata
Note that the source was found by google'ing. I am avoiding to be too lengthy and therefore only minimum amount of outputs are given. For necessary prediction only goals and dates are needed. I have always wondered whether it is useful to put into prediction as much statistical data as possible and it turns out that this must be avoided i.e. the right data for right prediction should be selected for best results. The CSV file should be first downloaded and a little-bit modified in order to use it as Mher Davtyan used it in his original example:
http://community.wolfram.com/groups/-/m/t/908804
then the path may be seen from the import function below:
oddsData =
SemanticImport[
"C:\\Users\\user\\Documents\\engsoccerdata-master\\data-raw\\italy3.\
csv", <|1 -> "Integer", 2 -> "String", 3 -> "Integer", 4 -> "String",
5 -> "String", 7 -> Automatic, 8 -> Automatic|>]
giving an output:
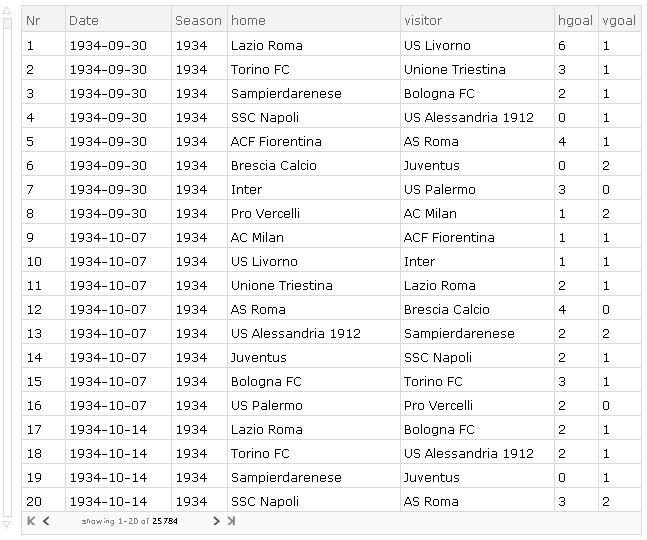
Yes, it consists of 25784 games. The collection of additions to Dataset are listed below and explained later:
scoreAdded =
oddsData[All,
Append[#,
"Score" ->
ToString[Slot["hgoal"]] <> ":" <> ToString[Slot["vgoal"]]] &]
homeAndAway =
scoreAdded[
Association["Home" -> GroupBy[#home &],
"Away" -> GroupBy[#visitor &]]];
data01 = (Flatten[(Transpose[{homeAndAway["Home",
scoreAdded[#]["home"], All, "Nr"] // Normal,
Thread[Rule[
homeAndAway["Home", scoreAdded[#]["home"], All,
"home"] // Normal,
homeAndAway["Home", scoreAdded[#]["home"], All,
"hgoal"] // Normal // Accumulate]]}] & /@
Range[1, 25784, 1]) // Union, 1] // Sort)[[All, 2]][[All,
2]];
data02 = (Flatten[(Transpose[{homeAndAway["Away",
scoreAdded[#]["visitor"], All, "Nr"] // Normal,
Thread[Rule[
homeAndAway["Away", scoreAdded[#]["visitor"], All,
"visitor"] // Normal,
homeAndAway["Away", scoreAdded[#]["visitor"], All,
"vgoal"] // Normal // Accumulate]]}] & /@
Range[1, 25784, 1]) // Union, 1] // Sort)[[All, 2]][[All,
2]];
data03 = (Flatten[(Transpose[{homeAndAway["Away",
scoreAdded[#]["visitor"], All, "Nr"] // Normal,
Thread[Rule[
homeAndAway["Away", scoreAdded[#]["visitor"], All,
"visitor"] // Normal,
homeAndAway["Away", scoreAdded[#]["visitor"], All,
"hgoal"] // Normal // Accumulate]]}] & /@
Range[1, 25784, 1]) // Union, 1] // Sort)[[All, 2]][[All,
2]];
data04 = (Flatten[(Transpose[{homeAndAway["Home",
scoreAdded[#]["home"], All, "Nr"] // Normal,
Thread[Rule[
homeAndAway["Home", scoreAdded[#]["home"], All,
"home"] // Normal,
homeAndAway["Home", scoreAdded[#]["home"], All,
"vgoal"] // Normal // Accumulate]]}] & /@
Range[1, 25784, 1]) // Union, 1] // Sort)[[All, 2]][[All,
2]];
addIndividualGoalsCumul[d_] :=
Join[d, Association["homeTeamHomeRating" -> (data01[[d["Nr"]]]),
"VisitorAwayRating" -> (data02[[d["Nr"]]]),
"homeTeamHomeConceded" -> (data04[[d["Nr"]]]),
"VisitorAwayConceded" -> (data03[[d["Nr"]]])]];
ratingsAdded = Map[addIndividualGoalsCumul[#] &, scoreAdded]
extractDate[a_] :=
Append[a, <|
"DateSuccessive" ->
FromDigits[StringSplit[a["Date"], "-"][[1]]] 100 - 190000 +
FromDigits[StringSplit[a["Date"], "-"][[2]]]|>]
dateNew = Map[extractDate[#] &, ratingsAdded]
addOutcome[a_Association] :=
If[a["hgoal"] == a["vgoal"], Append[a, Association["Outcome" -> 0]],
If[a["hgoal"] > a["vgoal"], Append[a, Association["Outcome" -> 1]],
Append[a, Association["Outcome" -> -1]]]]
outcomeAdded = Map[addOutcome[#] &, dateNew]
where data01, data02, data03 and data04 compute the cumulative scores and conceded scores for every team, the "DateSuccessive" modifies the "Date": 1934-06-23 to 03406 and 2017-05-28 to 11705 i.e. year and month, and "Outcome" is similar as in original post. The result table is:
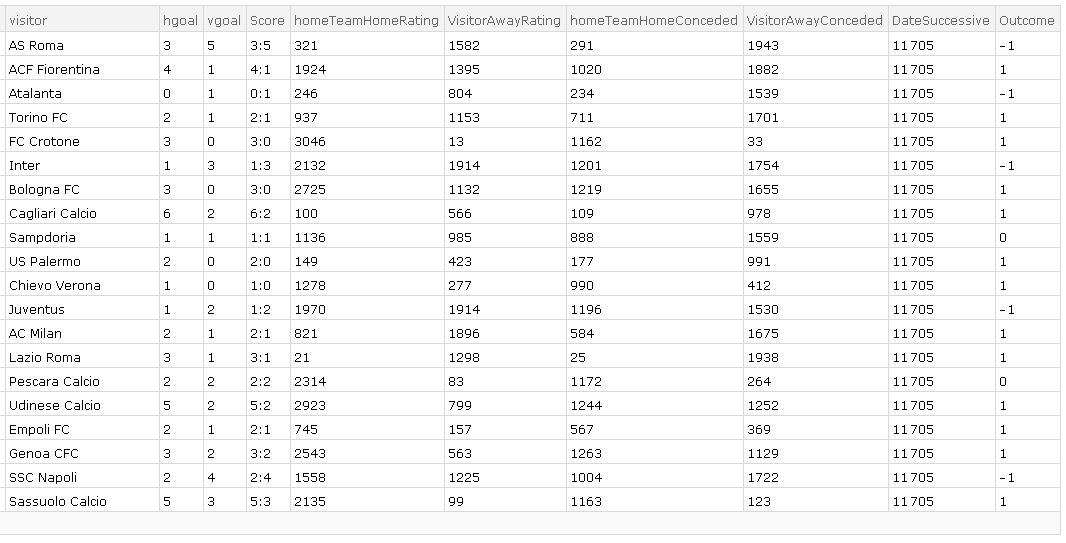
The test data starts from index:
In[96]:= Round[0.99218 Length[outcomeAdded]]
Out[96]= 25582
and consists of all 202 games played on yr 2017 for test set for Classifier:
Above we see the Testset and needed data using the code:
n = Round[0.99218 Length[outcomeAdded]];
{trainingSet, testSet} =
TakeDrop[outcomeAdded[
All, {"DateSuccessive", "homeTeamHomeRating", "VisitorAwayRating",
"homeTeamHomeConceded", "VisitorAwayConceded", "hgoal", "vgoal",
"Outcome"}], n];
c = Classify[trainingSet -> "Outcome", Method -> "LogisticRegression"] cm = ClassifierMeasurements[c, testSet -> "Outcome"]
with following results:
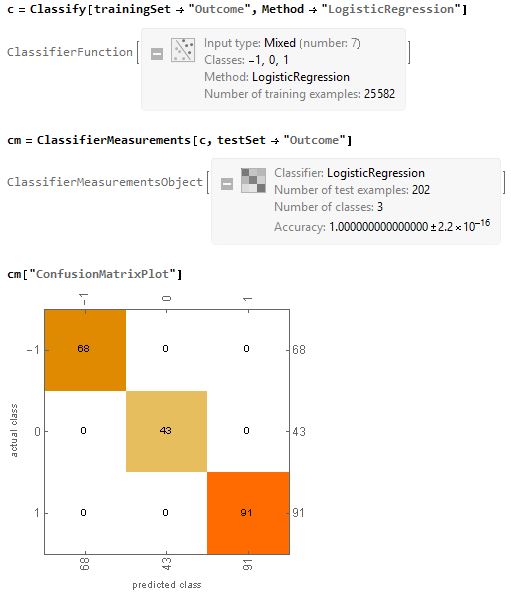
Visualisation:
In[138]:= actaulValue = testSet[All, "Outcome"] // Normal
Out[138]= {1, 1, -1, -1, 1, 1, 1, 0, -1, -1, 1, 1, 1, 1, 1, 0, 1, -1, \
0, -1, -1, 1, 1, 1, 0, 1, -1, -1, 1, 1, -1, 0, 1, 0, 0, 1, -1, 0, 1, \
-1, -1, 1, 0, 0, -1, 1, -1, 1, -1, 1, -1, -1, 1, 1, -1, -1, 1, -1, 1, \
-1, 1, 0, 1, 1, -1, -1, -1, 1, 1, 1, 0, -1, 1, -1, 1, -1, 0, -1, 1, \
0, -1, 0, 1, -1, 1, 0, -1, -1, 0, -1, 1, 0, 1, -1, 1, 1, 1, 1, -1, \
-1, -1, 1, 1, 0, 1, 1, 0, -1, -1, 1, -1, 1, 1, -1, -1, 1, -1, 0, -1, \
0, 0, -1, 0, 0, 1, -1, -1, 1, -1, 1, 0, 1, 1, -1, 0, 0, 1, 0, -1, 0, \
1, 0, 1, 1, -1, 1, 1, -1, -1, 0, 1, -1, 0, 0, 1, 1, 0, -1, -1, -1, 1, \
-1, 0, 1, 0, 1, 1, 1, -1, -1, 0, 0, 0, 1, 1, 1, 1, -1, 1, 1, 0, -1, \
-1, 1, -1, 1, 1, -1, 1, 1, 0, 1, 1, -1, 1, 1, 0, 1, 1, 1, -1, 1}
In[139]:= predictedValue =
c[Normal[testSet[[All, Values]]][[All, ;; 7]]]
Out[139]= {1, 1, -1, -1, 1, 1, 1, 0, -1, -1, 1, 1, 1, 1, 1, 0, 1, -1, \
0, -1, -1, 1, 1, 1, 0, 1, -1, -1, 1, 1, -1, 0, 1, 0, 0, 1, -1, 0, 1, \
-1, -1, 1, 0, 0, -1, 1, -1, 1, -1, 1, -1, -1, 1, 1, -1, -1, 1, -1, 1, \
-1, 1, 0, 1, 1, -1, -1, -1, 1, 1, 1, 0, -1, 1, -1, 1, -1, 0, -1, 1, \
0, -1, 0, 1, -1, 1, 0, -1, -1, 0, -1, 1, 0, 1, -1, 1, 1, 1, 1, -1, \
-1, -1, 1, 1, 0, 1, 1, 0, -1, -1, 1, -1, 1, 1, -1, -1, 1, -1, 0, -1, \
0, 0, -1, 0, 0, 1, -1, -1, 1, -1, 1, 0, 1, 1, -1, 0, 0, 1, 0, -1, 0, \
1, 0, 1, 1, -1, 1, 1, -1, -1, 0, 1, -1, 0, 0, 1, 1, 0, -1, -1, -1, 1, \
-1, 0, 1, 0, 1, 1, 1, -1, -1, 0, 0, 0, 1, 1, 1, 1, -1, 1, 1, 0, -1, \
-1, 1, -1, 1, 1, -1, 1, 1, 0, 1, 1, -1, 1, 1, 0, 1, 1, 1, -1, 1}
In[140]:= difference = actaulValue - predictedValue
Out[140]= {0, 0, 0, 0, 0, 0, 0, 0, 0, 0, 0, 0, 0, 0, 0, 0, 0, 0, 0, \
0, 0, 0, 0, 0, 0, 0, 0, 0, 0, 0, 0, 0, 0, 0, 0, 0, 0, 0, 0, 0, 0, 0, \
0, 0, 0, 0, 0, 0, 0, 0, 0, 0, 0, 0, 0, 0, 0, 0, 0, 0, 0, 0, 0, 0, 0, \
0, 0, 0, 0, 0, 0, 0, 0, 0, 0, 0, 0, 0, 0, 0, 0, 0, 0, 0, 0, 0, 0, 0, \
0, 0, 0, 0, 0, 0, 0, 0, 0, 0, 0, 0, 0, 0, 0, 0, 0, 0, 0, 0, 0, 0, 0, \
0, 0, 0, 0, 0, 0, 0, 0, 0, 0, 0, 0, 0, 0, 0, 0, 0, 0, 0, 0, 0, 0, 0, \
0, 0, 0, 0, 0, 0, 0, 0, 0, 0, 0, 0, 0, 0, 0, 0, 0, 0, 0, 0, 0, 0, 0, \
0, 0, 0, 0, 0, 0, 0, 0, 0, 0, 0, 0, 0, 0, 0, 0, 0, 0, 0, 0, 0, 0, 0, \
0, 0, 0, 0, 0, 0, 0, 0, 0, 0, 0, 0, 0, 0, 0, 0, 0, 0, 0, 0, 0, 0}
In[141]:= N@Normalize[Counts[Abs[difference]], Total]
Out[141]= <|0 -> 1.|>
Concluding that all yr 2017 game winners were correctly classified. Now, prediction with slightly larger set:
In[143]:= n = Round[0.99 Length[outcomeAdded]]
Out[143]= 25526
In[144]:= {trainingSetFull, testSetFull} = TakeDrop[outcomeAdded, n];
{trainingSet1,
testSet1} = {trainingSetFull[
All, {"DateSuccessive", "homeTeamHomeRating", "VisitorAwayRating",
"homeTeamHomeConceded", "VisitorAwayConceded", "hgoal", "vgoal",
"Outcome"}],
testSetFull[
All, {"DateSuccessive", "homeTeamHomeRating", "VisitorAwayRating",
"homeTeamHomeConceded", "VisitorAwayConceded", "hgoal", "vgoal",
"Outcome"}]};
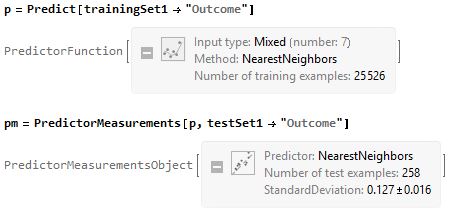
In[156]:= predictedValue =
Round[p[Normal[testSet1[[All, Values]]][[All, ;; 7]]], 1]
Out[156]= {1, 1, -1, -1, 1, 0, 1, -1, 1, -1, 1, 0, 1, -1, 1, 1, 0, 1, \
1, 1, -1, 1, 0, 1, 1, 0, 1, 1, -1, -1, 0, -1, 1, -1, -1, 1, 1, 1, 1, \
1, 0, 1, 0, 1, 1, -1, -1, 1, 1, 1, 1, 0, 0, 1, 0, 1, 1, 1, -1, -1, 1, \
1, 1, 0, -1, -1, 1, 1, 1, 1, 1, 0, 1, -1, 0, -1, -1, 1, 1, 1, 0, 1, \
0, -1, 1, 1, -1, 0, 1, 0, 0, 0, -1, 0, 1, -1, -1, 1, 0, 0, -1, 1, -1, \
1, -1, 1, -1, -1, 1, 1, -1, -1, 1, -1, 1, -1, 1, 0, 1, 1, -1, -1, -1, \
1, 1, 1, 0, -1, 1, -1, 1, -1, 0, -1, 1, 0, -1, 0, 1, -1, 1, 0, -1, \
-1, 0, -1, 1, 0, 1, -1, 1, 1, 1, 1, -1, -1, -1, 1, 1, 0, 1, 1, 0, -1, \
-1, 1, -1, 1, 1, -1, -1, 1, -1, 0, -1, 0, 0, -1, 0, 0, 1, -1, -1, 1, \
-1, 1, 0, 1, 1, -1, 0, 0, 1, 0, -1, 0, 1, 0, 1, 0, -1, 1, 1, -1, -1, \
0, 1, -1, 0, 0, 1, 1, 0, -1, -1, -1, 1, -1, 0, 1, 0, 1, 1, 1, -1, -1, \
0, 0, 0, 1, 1, 1, 1, -1, 1, 1, 0, -1, -1, 1, -1, 1, 1, -1, 1, 1, 0, \
1, 1, -1, 0, 1, 0, 1, 1, 1, -1, 1}
In[157]:= actaulValue = testSet1[All, "Outcome"] // Normal
Out[157]= {1, 1, -1, -1, 1, 0, 1, -1, 1, -1, 1, 0, 1, -1, 1, 1, 0, 1, \
1, 1, -1, 1, 0, 1, 1, 0, 1, 1, -1, -1, 0, -1, 1, -1, -1, 1, 1, 1, 1, \
1, 0, 1, -1, 1, 1, -1, -1, 1, 1, 1, 1, 0, 0, 1, 0, 1, 1, 1, -1, -1, \
1, 1, 1, 0, -1, -1, 1, 1, 1, 1, 1, 0, 1, -1, 0, -1, -1, 1, 1, 1, 0, \
1, -1, -1, 1, 1, -1, 0, 1, 0, 0, 1, -1, 0, 1, -1, -1, 1, 0, 0, -1, 1, \
-1, 1, -1, 1, -1, -1, 1, 1, -1, -1, 1, -1, 1, -1, 1, 0, 1, 1, -1, -1, \
-1, 1, 1, 1, 0, -1, 1, -1, 1, -1, 0, -1, 1, 0, -1, 0, 1, -1, 1, 0, \
-1, -1, 0, -1, 1, 0, 1, -1, 1, 1, 1, 1, -1, -1, -1, 1, 1, 0, 1, 1, 0, \
-1, -1, 1, -1, 1, 1, -1, -1, 1, -1, 0, -1, 0, 0, -1, 0, 0, 1, -1, -1, \
1, -1, 1, 0, 1, 1, -1, 0, 0, 1, 0, -1, 0, 1, 0, 1, 1, -1, 1, 1, -1, \
-1, 0, 1, -1, 0, 0, 1, 1, 0, -1, -1, -1, 1, -1, 0, 1, 0, 1, 1, 1, -1, \
-1, 0, 0, 0, 1, 1, 1, 1, -1, 1, 1, 0, -1, -1, 1, -1, 1, 1, -1, 1, 1, \
0, 1, 1, -1, 1, 1, 0, 1, 1, 1, -1, 1}
In[158]:= difference = Round[actaulValue - predictedValue, 0.01]
Out[158]= {0., 0., 0., 0., 0., 0., 0., 0., 0., 0., 0., 0., 0., 0., \
0., 0., 0., 0., 0., 0., 0., 0., 0., 0., 0., 0., 0., 0., 0., 0., 0., \
0., 0., 0., 0., 0., 0., 0., 0., 0., 0., 0., -1., 0., 0., 0., 0., 0., \
0., 0., 0., 0., 0., 0., 0., 0., 0., 0., 0., 0., 0., 0., 0., 0., 0., \
0., 0., 0., 0., 0., 0., 0., 0., 0., 0., 0., 0., 0., 0., 0., 0., 0., \
-1., 0., 0., 0., 0., 0., 0., 0., 0., 1., 0., 0., 0., 0., 0., 0., 0., \
0., 0., 0., 0., 0., 0., 0., 0., 0., 0., 0., 0., 0., 0., 0., 0., 0., \
0., 0., 0., 0., 0., 0., 0., 0., 0., 0., 0., 0., 0., 0., 0., 0., 0., \
0., 0., 0., 0., 0., 0., 0., 0., 0., 0., 0., 0., 0., 0., 0., 0., 0., \
0., 0., 0., 0., 0., 0., 0., 0., 0., 0., 0., 0., 0., 0., 0., 0., 0., \
0., 0., 0., 0., 0., 0., 0., 0., 0., 0., 0., 0., 0., 0., 0., 0., 0., \
0., 0., 0., 0., 0., 0., 0., 0., 0., 0., 0., 0., 0., 0., 0., 1., 0., \
0., 0., 0., 0., 0., 0., 0., 0., 0., 0., 0., 0., 0., 0., 0., 0., 0., \
0., 0., 0., 0., 0., 0., 0., 0., 0., 0., 0., 0., 0., 0., 0., 0., 0., \
0., 0., 0., 0., 0., 0., 0., 0., 0., 0., 0., 0., 0., 0., 0., 1., 0., \
0., 0., 0., 0., 0., 0.}
In[159]:= N@Normalize[Counts[Abs[difference]], Total]
Out[159]= <|0. -> 0.98062, 1. -> 0.0193798|>
showing 98 % accuracy.
10 Random Samples
randomMatches = RandomSample[testSetFull, 10]
Goals prediction is not so exact:
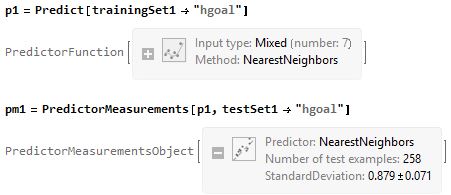
and for visiting team:
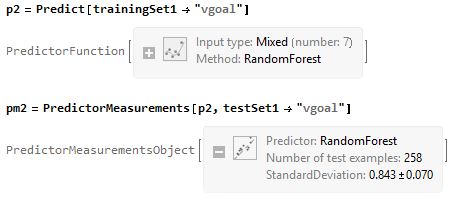
giving 60 % of accuracy.
Having "Outcome" correct, I will just show two tables of Predicted and Actual scores: 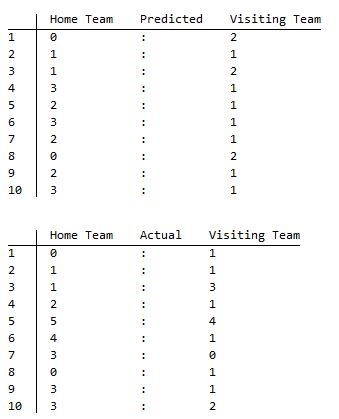
Conclusions
Clearly, game scores are harder to predict, almost 60 % are correct and 38 % differs with 1 goal. The predictor seems to work better when adding time-scale. Over the time the team's performance is changing and this must be reflected.