A one-week Wolfram U Daily Study Group covering Stephen Wolfram's best-selling book What is ChatGPT Doing... and Why Does It Work? begins on Monday, March 3, 2025.
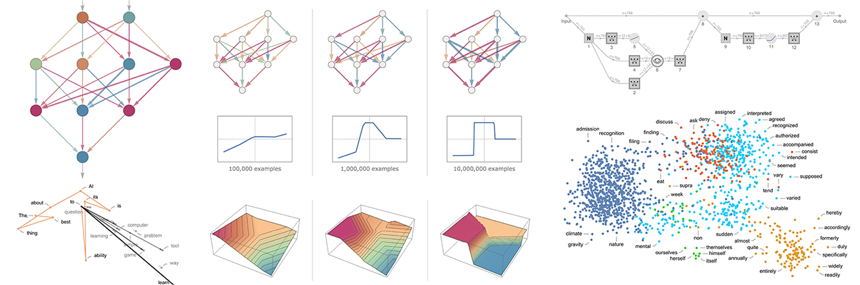
Join a cohort of fellow learners to discover the principles that underly ChatGPT and other LLMs. I have adapted the material from the aforementioned book into a series of four notebooks covering topics ranging from probabilistic text generation to neural nets, machine learning, embeddings and even transformer models.
On the final day of the study group, I will go through a bunch of interesting examples using our new Notebook Assistant, which closely integrates powerful LLMs with the Wolfram documentation system and notebook interface.
Stephen Wolfram's book is aimed at anybody who is curious about these ideas, and this study group follows the book's lead. Therefore, no prior Wolfram Language, machine learning, or even coding experience is necessary to attend this study group.
Please feel free to post any questions, ideas and/or useful links in this thread between sessions—we always love to continue the discussion here on Community! If you'd like to read the discussion from the last time we ran this study group, you can find that here.
This is a one-week study group that will run from March 3 through March 7 2025 at 11:00am Central US Time each day.
REGISTER HERE
