Punctuation Restoration With Recurrent Neural Networks
Mengyi Shan, Harvey Mudd College, mshan@hmc.edu
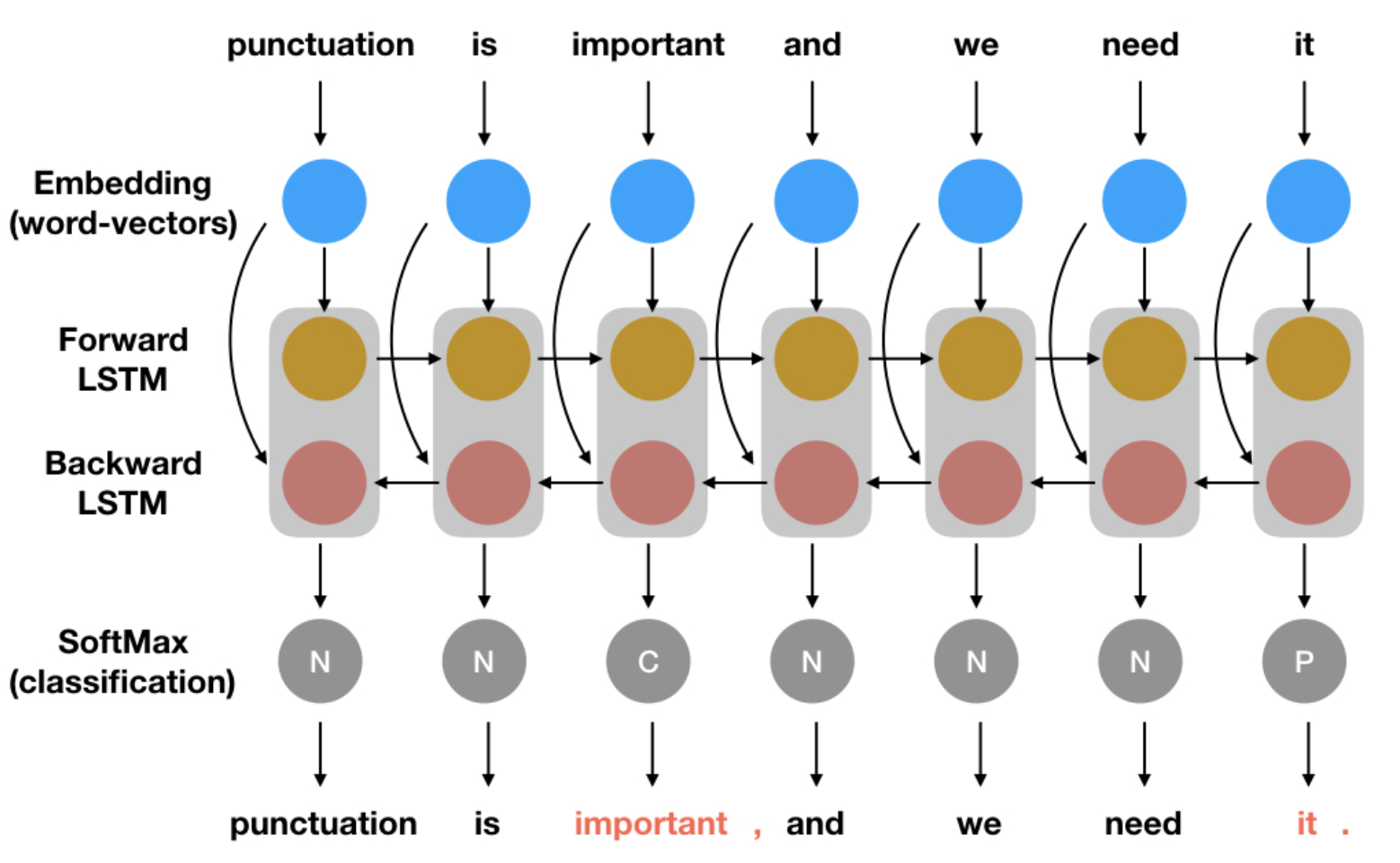
All codes posted on GitHub: https://github.com/Shanmy/Summer2018Starter/tree/master/Project. Raw results in the attached notebook.
Introduction
In natural language processing problems such as automatic speech recognition (ASR), the generated text is normally unpunctuated, which is hard for further recognition or analysis. Thus punctuation restoration is a small but crucial problem that deserves our attention. This project aims to build an automatic "punctuation adding" tool for plain English text with no punctuation.
Since the input text could be considered as a sequence in which context is important for every single word's properties, recurrent characteristics of neural networks are considered to be a good method. Traditional approaches to this problem include usage of various recurrent neural networks (RNN), especially long short-term memory layers (LSTM). This project examines several models built from different layers and introduces bidirectional operators which can significantly improve the result compared with old methods.
Methods
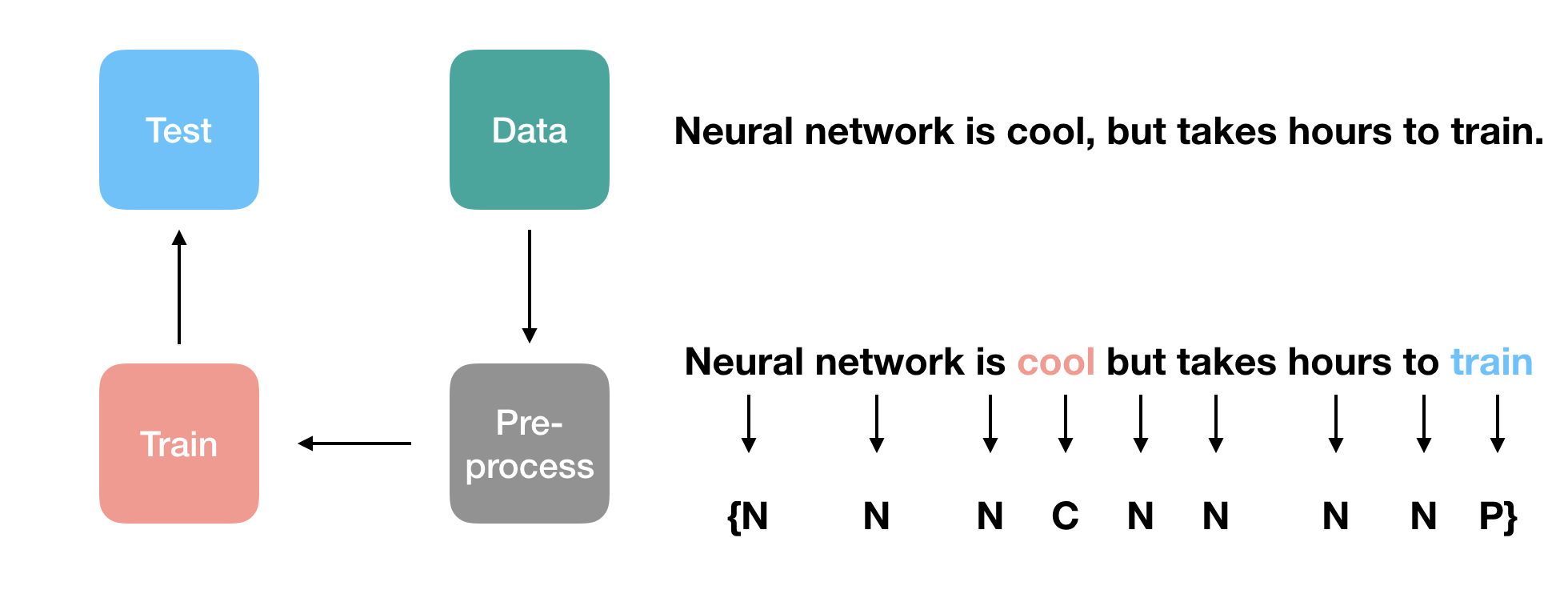
There're four basic steps in the whole process. First, we get the corpus of articles (with punctuations). Then, we keep the periods and commas in the corpus but change the question marks, exclamation marks, and colons to periods and commas, while removing all other punctuations. With this pure text, we tag each word as one of {NONE, COMMA, PERIOD} by judging if it is followed by a punctuation or not. And this set of tagging rules are sent to a neural network model for training. Finally, we test the result on another piece of articles, which is the test set.
Data
Basically, we have two pieces of data. The first one is the Wikipedia text of 4000 nouns (deleting missing), and the second is 50 novels from Wolfram data repository.
(*Get wikipedia text of 4000 nouns*)
nounlist = Select[WordList[], WordData[#, "PartsOfSpeech"][[1]] == "Noun" &];
rawData = StringJoin @@ DeleteCases[Flatten[WikipediaData[#] & /@ Take[nounlist, {1, 4000}], 2], _Missing]
(*Get text of 50 novels*)
books = StringJoin @@ Get /@ ResourceSearch["novels", 50];
Pre-processing
The first goal of the preprocessing step is to purify the text. That is, since we only consider commas and periods, we should either delete or replace other characters and punctuations. Also, for convenience, all numbers are replaced with 1 first. All other characters are removed from the text.
(*Show sets of characters replaced with comma, period, whitespace, one and null respectively*)
toComma = Characters[":;"];
toPeriod = Characters["!?"];
toWhiteSpace = {"-", "\n"};
toOne = {"0", "1", "2", "3", "4", "5", "6", "7", "8", "9"};
toNull[x_String] := Complement[Union[Characters[x]], ToUpperCase@Alphabet[], Alphabet[], toOne, toComma, toPeriod, toWhiteSpace, {".", ",", " "}];
Then complete the replacement and modify it to pure form. And we include a validation test to examine its purity.
(*Replacement and modification. End with lowercase text with only periods, alphabets and commas.*)
toPureText[x_String] :=
StringReplace[#, ".," .. -> ". "] &@
StringReplace[#, ". " .. -> ". "] &@
StringReplace[#, {" ," -> ",", " ." -> "."}] &@
StringReplace[#, {"1" .. -> "one", " " .. -> " "}] &@
StringReplace[#, {"1. 1" -> "", "1, 1" -> "1"}] &@
StringReplace[#, {" " .. -> " "}] &@
StringReplace[#, {"," -> ", ", "." -> ". "}] &@
ToLowerCase@
StringReplace[{toComma -> ",", toPeriod -> ".", toNull[x] -> "",
toWhiteSpace -> " ", toOne -> "1"}][x];
(*validation test*)
VerificationTest[Length@StringSplit@x == Length@TextWords@x]
Then we define a function fPuncTag that can generate the corresponding tagging given a piece of text with punctuation.
(*Define the tagging function, and maps it to original text. Original text is partitioned into pieces of 200 words*)
fPuncTag := Switch[StringTake[#, -1], ".", "a", ",", "b", _, "c"] &;
fWordTag[x_String] := Map[fPuncTag, Partition[StringSplit[x], 200], {2}];
And we can thus remove the punctuation, and build a set of rules between the unpunctuated text and the generated tagging.
fWordText[x_String] := StringReplace[#, {"," -> "", "." -> ""}] & /@ StringRiffle /@ Partition[StringSplit[x], 200];
fWordTrain[x_String] := Normal@AssociationThread[fWordText[x], fWordTag[x]];
totalData = fWordTrain@toPureText@rawText
With the total data, we want to divide it into three groups: the training set, the validation set, and the test set.
(* First we know that the length is 63252, then we divide it by 15:3:1*)
order = RandomSample[Range[63252]];
trainingSet = totalData[[Take[order, 50000]]];
validationSet = totalData[[Take[order, {50001, 60000}]]];
testSet = totalData[[Take[order, {60001, -1}]]];
Train
During neural network training, I used 8 different combinations of layers, out of which 4 are worth considering. They are listed as followed. LSTM layer, gate recurrent layer, and basic recurrent layer are three types of recurrent layers, each representing a net that takes a sequence of vectors and outputs a sequence of the same length. LSTM is commonly used in natural language processing problems, so we start with it as a penetrating point.
(*Pure LSTM*)
net1 = NetChain[{
embeddingLayer,
LongShortTermMemoryLayer[100],
LongShortTermMemoryLayer[60],
LongShortTermMemoryLayer[30],
LongShortTermMemoryLayer[10],
NetMapOperator[LinearLayer[3]],
SoftmaxLayer["Input" -> {"Varying", 3}]},
"Output" -> NetDecoder[{"Class", {"a", "b", "c"}}]
];
(*Gate Recurrent*)
net2 = NetChain[{
embeddingLayer,
LongShortTermMemoryLayer[100],
GatedRecurrentLayer[60],
LongShortTermMemoryLayer[30],
GatedRecurrentLayer[10],
NetMapOperator[LinearLayer[3]],
SoftmaxLayer["Input" -> {"Varying", 3}]},
"Output" -> NetDecoder[{"Class", {"a", "b", "c"}}]
];
(Basic Recurrent)
net3 = NetChain[{
embeddingLayer,
LongShortTermMemoryLayer[100],
BasicRecurrentLayer[60],
LongShortTermMemoryLayer[30],
BasicRecurrentLayer[10],
NetMapOperator[LinearLayer[3]],
SoftmaxLayer["Input" -> {"Varying", 3}]},
"Output" -> NetDecoder[{"Class", {"a", "b", "c"}}]
];
(*Bidirectional*)
net4 = NetChain[{
embeddingLayer,
LongShortTermMemoryLayer[100],
NetBidirectionalOperator[{LongShortTermMemoryLayer[40],
GatedRecurrentLayer[40]}],
NetBidirectionalOperator[{LongShortTermMemoryLayer[20],
GatedRecurrentLayer[20]}],
LongShortTermMemoryLayer[10],
NetMapOperator[LinearLayer[3]],
SoftmaxLayer["Input" -> {"Varying", 3}]},
"Output" -> NetDecoder[{"Class", {"a", "b", "c"}}]
];
The embedding layer is used to change words into vectors that represent their semantic characteristics.
(*The embedding layer here*)
embeddingLayer = NetModel["GloVe 100-Dimensional Word Vectors Trained on Wikipedia and Gigaword 5 Data"]
With all those neural network models set up, we can train each neural network. To save time, I first trained all models with a small data set of only 3 million words to compare their behaviors.
(*train the neural network while saving the training object*)
NetTrain[net, trainingSet, All, ValidationSet -> validationSet]
Test
Since this classification problem is a problem of a skewed dataset, that is, most of the words should have the tag "None", it doesn't make sense to use "accuracy" to measure the models' behavior. Even if it simply do nothing and always return "None", it will have a high accuracy that is the percentage of "None" in the whole tagging set. Instead, to evaluate the behavior of the models, we introduce the concept of precision, recall, and f1-score.
(*Precision and recall*)
precision = truePrediction/allTrue
recall = truePrediction/allPrediction
F1 = HarmonicMean[{precision, recall}]
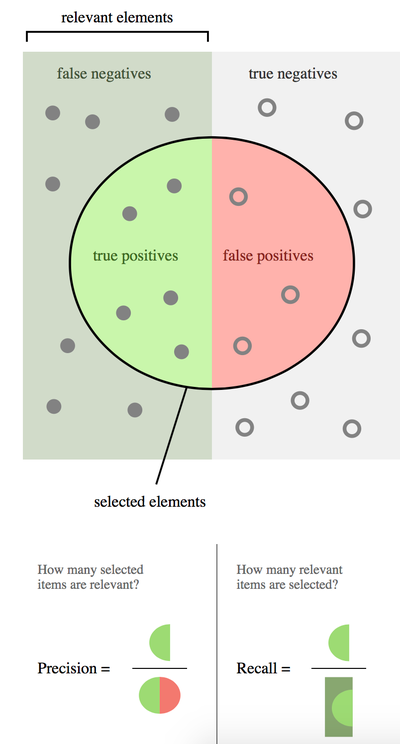
For a given test set, first, we want to remove its punctuations and run the trained model on it.
(*romve punctuation and run the model*)
noPuncTest = Keys /@ testSet
result = net["TrainedNet"] /@ noPuncTest;
Then we changed the tags to 1,2 and 0. And we calculate the elementwise product of realTag and resultTag. If an element is 4, it means that both the realTag and resultTag is 2, which counts as a successful prediction of a comma. An element of 2 represents a successful prediction of a period.
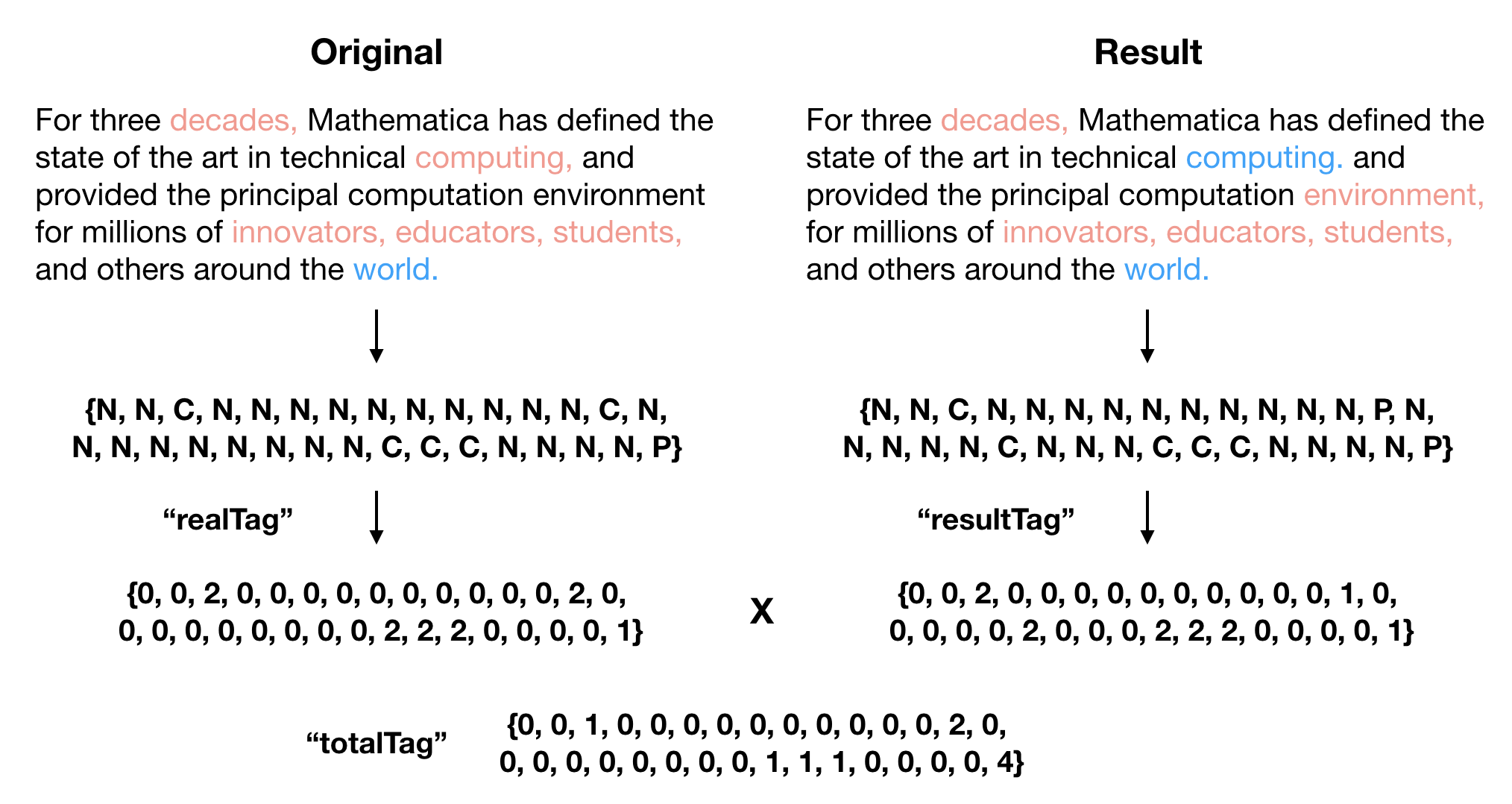
(*Change the tags to numerical values and count 1s and 4s*)
realTag = Replace[Flatten[Values /@ Take[testSet, 3252]], {"a" -> 1, "b" -> 2, "c" -> 0}, {1}];
resultTag = Replace[Flatten[result], {"a" -> 1, "b" -> 2, "c" -> 0}, {1}];
totalTag = realTag*resultTag;
Now we can use totalTag, resultTag, and realTag to calculate precision, recall, and f1-score.
(*Precision*)
PrecPeriod = N@Count[totalTag, 1]/Count[resultTag, 1]
PrecComma = N@Count[totalTag, 4]/Count[resultTag, 2]
(*Recall*)
RecPeriod = N@Count[totalTag, 1]/Count[realTag, 1]
RecComma = N@Count[totalTag, 4]/Count[realTag, 2]
(*F1*)
F1Period = (2*RecPeriod*PrecPeriod)/(RecPeriod + PrecPeriod)
F1Comma = (2*RecComma*PrecComma)/(RecComma + PrecComma)
Result
Ten neural networks are trained based on a small dataset with different layers. Only using Long Short-Term Memory layers gives an f1 score of 13% and 11% for periods and commas. Introducing dropout parameters, pooling layers, elementwise layers, basic recurrent layers and gate recurrent layers all produce an f1 score between 10% and 30%, showing no significant improvement. Introduction of the bidirectional operator (combining two recurrent layers) improves the scores to 53% and 47%, and to 72% and 60% respectively when training on a larger dataset of 10M words.
Here are the results for the three different neural networks trained with a 3M small dataset, and bidirectional neural network (which has the best performance in the small dataset) trained with a larger dataset of 10M words. The first figure is of the period and the second is of the comma.
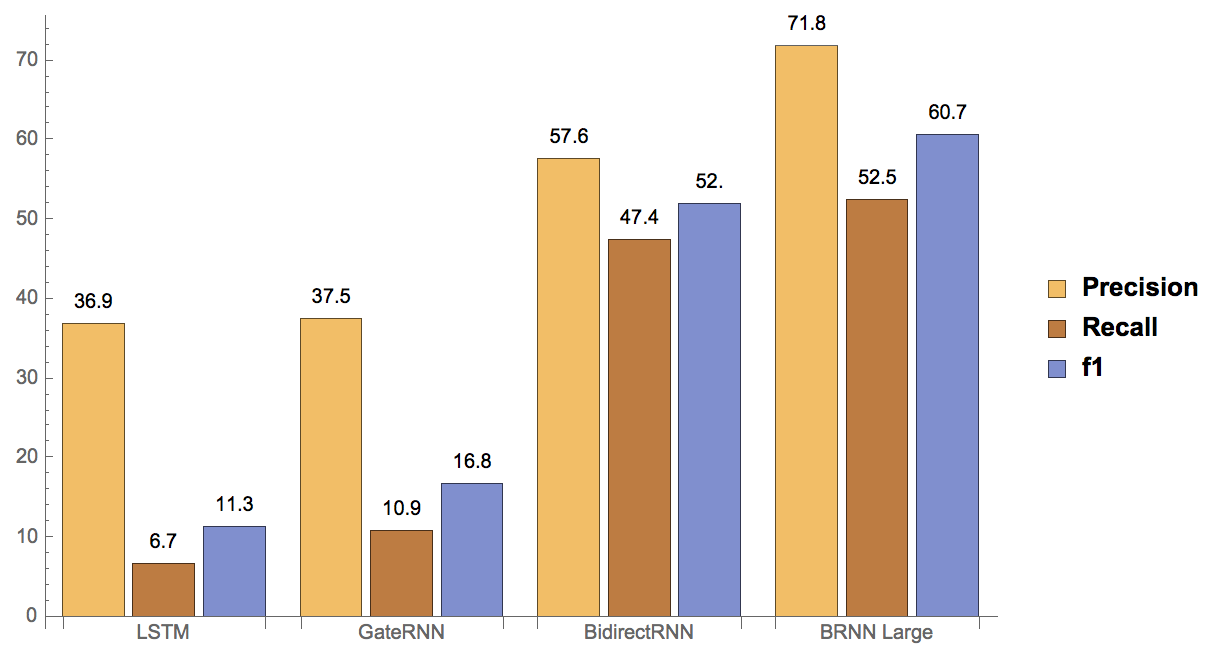
We can easily observe the advantage of the bidirectional operator in terms of both periods and commas, precision and recall. Instead of the sequence to sequence learning, "tagging" is a significantly more efficient and accurate way to restore punctuation in plain text. Since every words' tags ("None", "Comma", "Period") is influenced by its context, it makes sense that recurrent neural networks and bidirectional operators show great potential in this research.
Generally, the recall score is significantly lower than the precision score, suggesting that the model generates too many punctuations than it should. This could be due to the dataset of Wikipedia which is not clean enough. In the Wikipedia text, sometimes there're equations, translations, or other strange characters that we simply delete. This changed the ratio of punctuations to words and produces some segments of text that is "full of" punctuations since all words are not recognized and simply deleted. One example of those "not clean segment" is shown below.
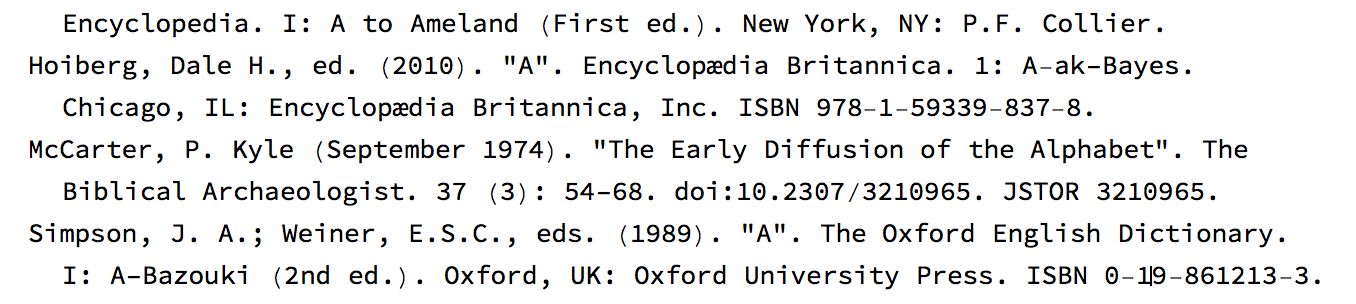
Also, the overall performance on commas is slightly worse than on periods. This also makes sense from a linguistics point of view. There seems to be a concrete linguistics set of rules for the period, but the usage of comma greatly depends on personal writing style. For example, you could say either "I like apples but I don't like bananas.", or "I like apples, but I don't like bananas." In this way, it's really hard to build a model for comma prediction with such high accuracy. But fortunately, sometimes adding commas or not doesn't really influence the overall meaning of the sentence. So it's okay to be tolerant to a slightly worse performance on commas.
Future Works
70% f1-score is still not enough for the application. Planned future work focuses on improving accuracy to a level suitable for usage in industry. The most urgent and important future work is using a larger data size. We can observe great improvement when changing from 3M to 10M dataset, but it's still far less than enough.
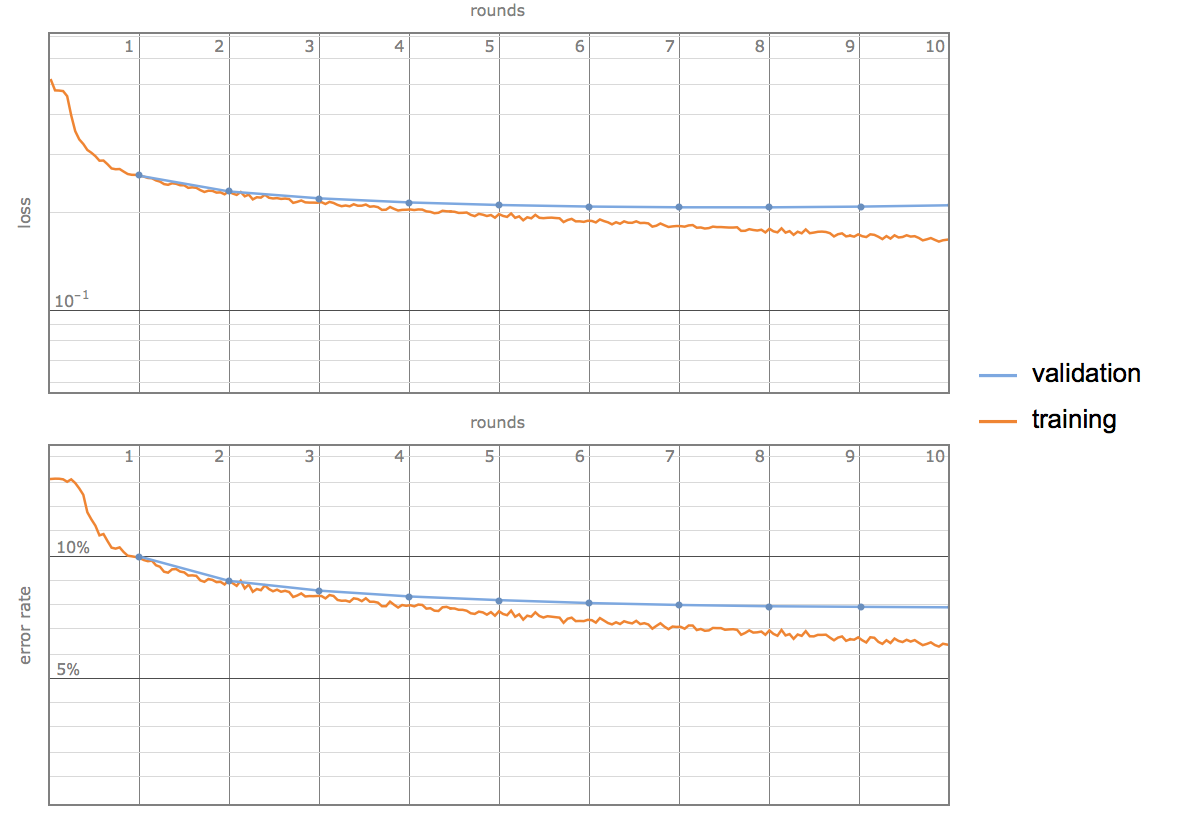
If we take a closer look at the evolution plots during training, we can see that the error rate and loss of training set are continuously decreasing, while the error rate and loss of the validation set soon reaches a stable state and doesn't change too much. The gap between those two curves suggests the possibility of overfitting, and it should greatly help if we introduce better and more data.
Also, punctuation restoration should not be limited to periods and comma. A more rigorous study of the question mark, exclamation mark, colon, and quotation mark is expected. However, we should note that the choice of most punctuations is not restricted to one possibility. In cases like distinguishing a period with an exclamation mark, we cannot expect a high f1-score. But it's still an interesting topic, may be useful for topics like sentimental analysis.
Acknowledgement
I would like to thank the summer school for providing the environment and background skills for me to finish this project. Especially, I want to thank my mentor for helping me with neural network problems and debugging.
Data and Reference
- Wolfram Data Repository
- Wikipedia
- Tilk O, et al. "Lstm for Punctuation Restoration in Speech Transcripts." Proceedings of the Annual Conference of the International Speech Communication Association, Interspeech, 2015-January, 2015, pp. 683\687.
Attachments: