Here is a Min-Heap package I wrote for my WSS project, Optimal Packing of Bidisperse Circles . There were 2 main challenges in this implementation: dealing with in-place modification of function arguments, and implementing the equivalent to a pop() operation, extracting in-place the last element of the list without copying the whole list.
I stored each heap (balanced binary tree) in a list, where each node at position i has children nodes at positions $2i$ and $2i+1$, and parent node at Floor[ $\frac{i}{2}$]. A Min-Heap satisfies the Min-Heap property: the value at each node is strictly less than or equal to each value at its children nodes.
I wrote 6 functions for this package (and another 6 for the Project-specific version): MinHeapQ, HeapifyUp, HeapifyDown, HInsert, HExtractMin and HBuild. Here are their descriptions:
MinHeapQ
This function tests if the list argument is a MinHeap or not, in $O(n)$ tests.
MinHeapQ[heap_List]:=And@@Table[heap[[Floor[i/2]]]<= heap[[i]], {i, 2, heap//Length}];
HeapifyUp
This function corrects a single error at index $i$ in the Min-Heap, recursively swapping it with its parent node until the Min-Heap property is satisfied. The HeapifyUp operation is asymptotic $O(\log n)$ complexity, given that it in the worst case traverses a whole branch of the tree, from a leaf upwards.
HeapifyUp:=Function[{heap, i}, Which[
(*at root*)
i==1,
heap,
(*satisfies minHeap prop, return*)
heap[[i]]>= heap[[Floor[i/2]]],
heap,
(*if parent greater, swap with parent, and recursively call at new position*)
heap[[i]]<= heap[[Floor[i/2]]],
{heap[[i]], heap[[Floor[i/2]]]}={heap[[Floor[i/2]]], heap[[i]]};
HeapifyUp[heap, Floor[i/2]];
],
HoldFirst
]
To deal with the in-place modification issue, I wrapped the Which cases in Funtion[, HoldFirst], allowing the heap list to be passed Unevaluated.
HeapifyDown
In an analogous manner, HeapifyDown corrects a violation at position $i$, comparing with child nodes and swapping with the smaller of the 2 (or 1, or not swapping) if its value is less than $i$'s value. The HeapifyDown operation is also asymptotic $O(\log n)$ complexity, through the same explanation above.
HeapifyDown:=Function[{heap, i}, With[{n = heap//Length}, Which[
(*at leaf*)
n< 2i,
heap,
(*only left child exists, swap*)
n<=2i && heap[[i]]>= heap[[2i]],
{heap[[i]], heap[[2i]]}={heap[[2i]], heap[[i]]};
HeapifyDown[heap, 2i];,
(*only left child exists, property satisfied*)
n<=2i && heap[[i]]<= heap[[2i]],
heap,
(*property satisfied*)
heap[[i]]<= heap[[2i]] && heap[[i]]<= heap[[2i+1]],
heap,
(*swap with left child*)
heap[[i]]>=Min[heap[[2i]], heap[[2i+1]]] && heap[[2i]]<= heap[[2i+1]],
{heap[[i]], heap[[2i]]}={heap[[2i]], heap[[i]]};
HeapifyDown[heap, 2i];,
(*swap with right child*)
heap[[i]]>= Min[heap[[2i]], heap[[2i+1]]] && heap[[2i]]>heap[[2i+1]],
{heap[[i]], heap[[2i+1]]}={heap[[2i+1]], heap[[i]]};
HeapifyDown[heap, 2i+1];
] ],
HoldFirst
]
HInsert
This function inserts an element into the heap, by appending it to the end of the heap and applying HeapifyUp into the element is at its correct position in the heap (and overall, the heap satisfies the Min-Heap property again). The insert operation has $O(\log n)$ asymptotic complexity.
HInsert:=Function[{heap, element},
AppendTo[heap, element];
HeapifyUp[heap, heap//Length];,
HoldFirst
]
HBuild
HBuild builds a heap out of a list in an in place manner, by applying HeapifyDown to all non-leaf nodes. It can be shown that the complexity of this operation is $O(n)$, linear in input size, not $O(n \log n)$, as follows: At height $k$, the number of nodes is given by $2^k$. We can thus write the total complexity as the sum $$ T = \sum_{k=0}^h (h-k)2^k $$ where $h = Log_2 n$ is the height of the tree. We can evaluate this sum in Mathematica:
In[2]:= Sum[(Log2[n] - k) 2^k, {k , 0, Log2[n]}]
Out[2]= (-2 Log[2] + 2 n Log[2] - Log[n])/Log[2]
Which is clearly $O(n)$. As for the implementation, here it is:
BuildH:=Function[{list},
Map[HeapifyDown[list, #]&, Range[Floor[(list//Length)/2], 1, -1]];,
HoldFirst
]
HExtractMin
HExtractMin was by far the hardest part of this package, and definitely one of the hardest in my project. This function should return the minimum element in the heap, a.k.a heap[[1]], delete it from the heap, while maintaining the Min-Heap property. The traditional algorithm for this procedure is as follows:
- Swap the first element and the last element
- Pop the last element
- HeapifyDown the first element to correct Min-Heap Property
However, there is no pop operation in the WL. There are functions like Drop, Take and Span, but they all copy the list, so have $O(n)$ complexity and thus are too slow. The Drop operation on a $10^6$ element list runs $10$ times slower than the HeapifyDown operation! I addressed this issue with a simple amortization algorithm:
The algorithm will require a global counter dcount which counts the number of deletion operations (initialized internally at 0). The procedure:
Every time extractMin is performed, the counter is incremented and a null element [Infinity] is left at the bottom (maintaining heap size) . After $n$ such operations, the asymptotic cost of a Select operation is $O(n)$, making the amortized cost of the "pop" $O(1)$. In a $10^6$ element list, the Select operation takes about 1 second, and happens every $5. 10^5$ deletes. Clearly, the amortized cost is efficient as is, but can still be tuned in the package as needed. I arbitrarily choose half the size to correspond to (roughly) the size of a next layer of leaves, all infinity (but, of course, the infinities aren't all at the leaves).
Anyway, here is the implementation:
HExtractMin:=Function[{heap}, Block[ {n = heap//Length, x = heap[[1]]},
(*increment delete counter*)
dcount++;
(*swap last element*)
{heap[[1]], heap[[n]]}={heap[[n]], heap[[1]]};
(*set infinity at the end*)
heap[[n]] = Infinity;
(*Amortization case*)
If[dcount > n / 2,
dcount = 0;
heap = Select[heap, #!= Infinity&];
BuildH[heap];
];
(*Corrects swapped position*)
HeapifyDown[1];
(*output minimum*)
x
],
HoldFirst
]
HeapifyDown Test
To conclude this post, I ran a couple tests on HeapifyDown up to where my memory could hold:
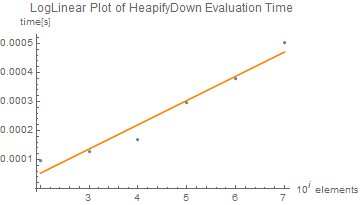
And, as we can see, the evaluation time is quite linear, and pretty fast.
If any optimizations or better ExtractMin algorithms are found, send me them! Also, checkout this implementation of a Augmented Min-Heap I wrote for my whole project (in the notebook at the end).
Attachments: