Introduction
The Convolution-based approach described a high quality and performant method to perform ASCII art. In that approach, convolution was used, which brought up a necessity to consider more parameters. A new solution was to develop a classifier that would predict the character choices to automate the process; that is why this approach is called the Classifier-based approach.
Approach
This approach is about subdividing the image into a rectangular grid and mapping each cell to an ASCII character glyph. The classifier trained by the Convolution-based approach performs mapping with a decision tree.
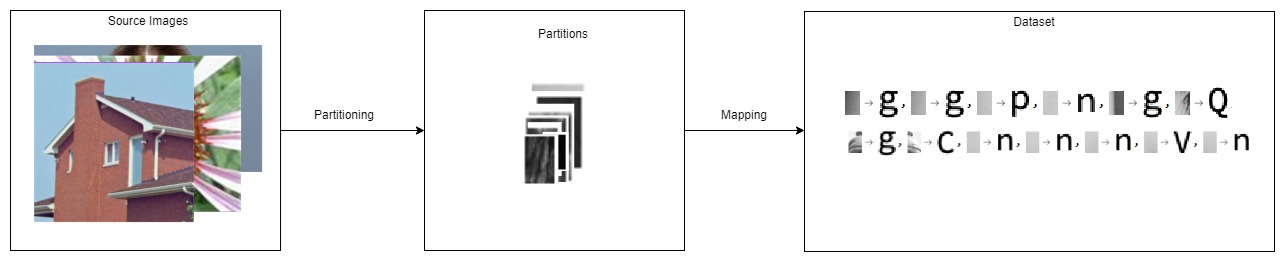
Creating a dataset is achieved by partitioning the images. Each partition size is the same as the character raster glyphs. Character glyphs have a dimension of 15x24. After that, the Convolution-based approach was applied to each partition to find a representative ASCII character. Below it is seen how a sample generated dataset looks like:
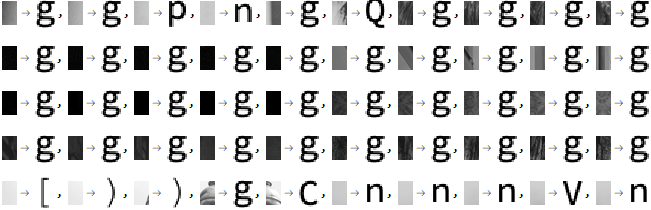
The generated dataset includes the partitions from 200 HD images, which leads to approximately 850000 training samples. To train a classifier, we use:
classifierFunction = Classify[dataset, Method -> "DecisionTree"]
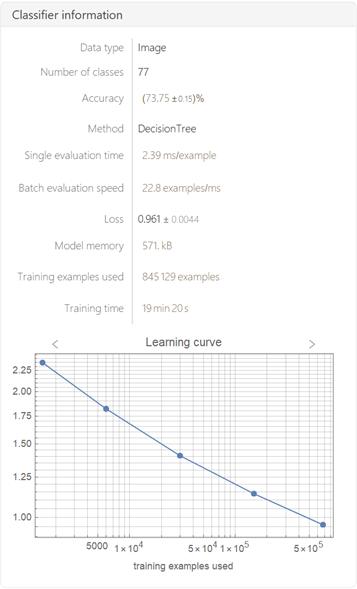
Here is an example to show how the classifier is operating:
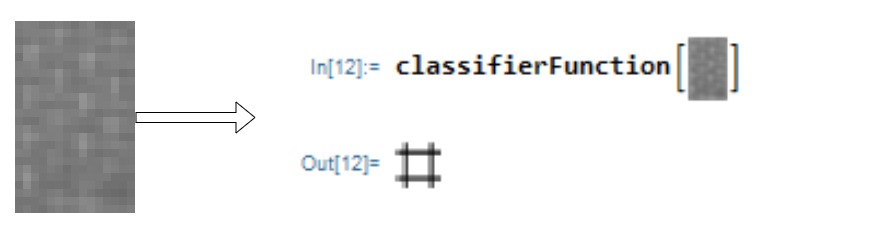
It is worth mentioning that 850000 training samples were not enough to classify all 95 characters; a more comprehensive dataset will solve it.
As we now have a trained classifier, let us look at how to achieve the ASCII art. First of all, we apply minor preprocessing on the image to highlight some features:
ImageAdjust[HistogramTransform[RemoveAlphaChannel[image]]]
The experimental analysis showed that this preprocessor is most suited for a feature highlighting. As image is prepared we partition it by the size of character rasters:
partitionSize =
ImageDimensions[Information[classifierFunction, "Classes"][[1]]];
partitions = ImagePartition[image, partitionSize];
Now we feed this data into our classifier and assemble the resulting image:
imageData = classifierFunction[#] & /@ partitions;
ImageAssemble[imageData]
Results
Below are a few results to demonstrate the function behavior:
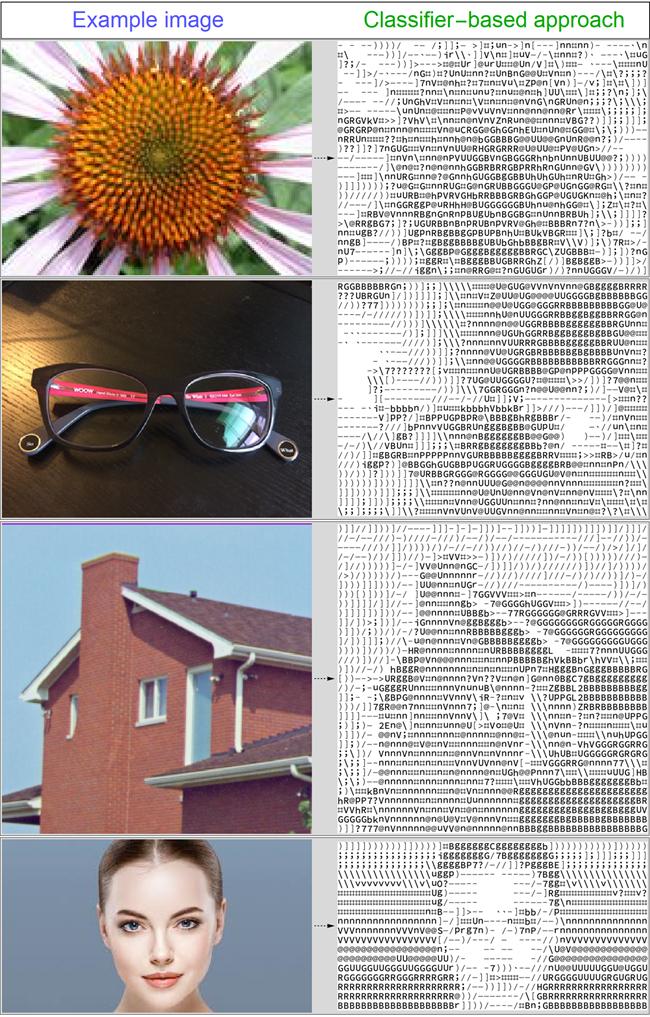
character rasters have {15, 24}
dimensions, resizing the input image to match 800
in one size
Let us now compare this approach with the Convolution-based approach both visual and performance vise:
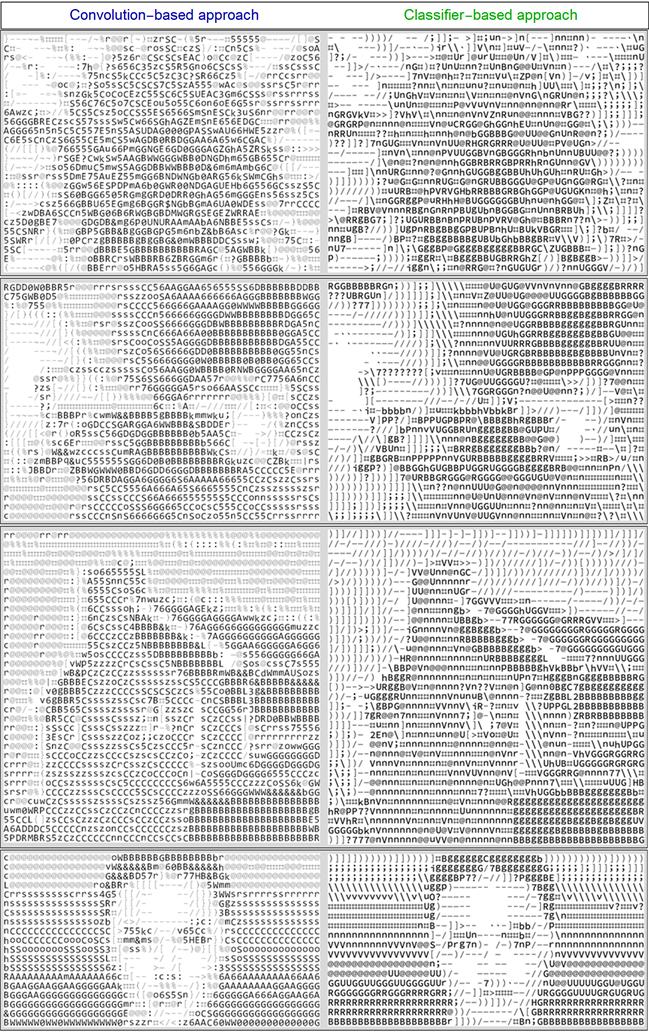
character rasters have {15, 24}
dimensions, resizing the input image to match 800
in one size
And the time complexity:
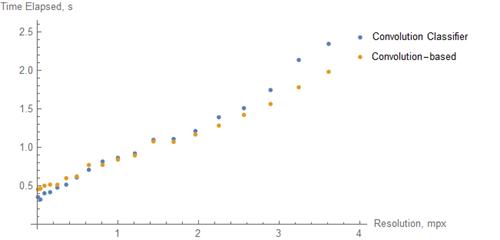
Conclusion
As we can see, the performance is nearly identical in both speed and visual vise. However, the Classifier-based approach has a significant flaw: the limited quantity of character font size. For every new font size, one needs to train a new classifier. However, suppose one needs to perform ASCII art with limited quantity character sizes. In that case, the Classifier-based approach will show a more consistent result. In conclusion, the Convolution-based approach proved itself excellent performing, so in general, considering the issues, there is no point in training a classifier.
References & Credits
Thanks to Wolfram Team and Mikayel Egibyan in particular for mentoring the internship.
Attachments: