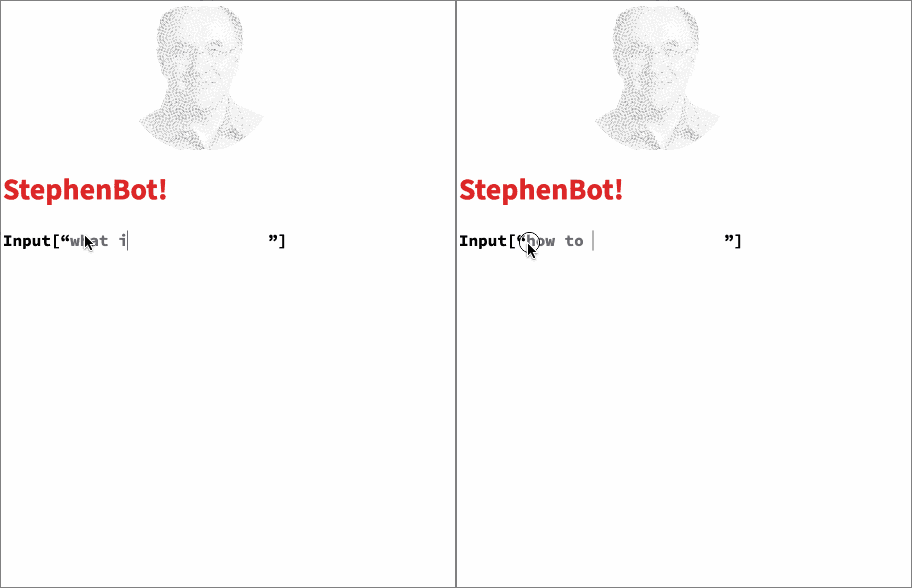
Hello, Wolfram Community!
I’ve developed StephenBot to honor Stephen Wolfram's innovations and his significant influence in computational science and technology. It celebrates his dedication to public education and his commitment to sharing his knowledge with the world. StephenBot has access to Stephen’s public presentations, writings and live streams.
You can now head over to
and ask anything, from his first computer or favourite movie to his ideas on Observer Theory and Ruliad, or his current understanding of the Second Law of Thermodynamics!
I'm happy to answer your questions and would love to get everyone’s feedback.
If you're interested in the technical details, read on!
How does it work? Context is all you need!
LLMs, trained on large amounts of data (public or private), have a general understanding of patterns in natural language and are trained to produce convincing, human-like responses. However, due to their lack of deep understanding like humans, and limitations and biases in their training data, they often suffer from what’s now the 2023 word of the year, “hallucinate”! It refers to generating information that is incorrect, irrelevant or nonsensical. So, any useful application of LLMs needs more than just prompt engineering.
Suppose you want your LLM to answer questions based on a specific PDF or Word document. You can now upload that to GPT-4 or copy-paste the content into ChatGPT and ask questions about the document. If the content is small enough, ChatGPT will remember it and, based on the given document, attempt to answer your questions. This given data is now part of the context.
Context is the information embedded in the prompt for the LLM to draw from and have a conversation with you. Normally, the context contains all previous messages (yours and the AI’s) in a single chat session.
Now, imagine your PDF or Word document is quite large, or you have hundreds of documents you want the LLM to remember and answer questions based on. You can't copy & paste all the content into the prompt box. It won’t remember all of that as it can only accept a limited number of characters (or tokens, to be technically correct). This is known as context size limitations. To deal with these limitations, we need to include only relevant documents based on the user's question. We need a retrieval mechanism to find, rank and package only the most relevant parts of the documents into the interactions with the LLM.
Context Retrieval
Context retrieval is the classic problem of data retrieval in computer science and NLP, where techniques from TF-IDF to similarity matching based on vector embeddings have been developed to find the most relevant information or document for a given query. When applied to LLMs, it’s often referred to as “Retrieval-Augmented Generation” or RAG, aiming to “augment” the LLM's context with relevant, specific information or otherwise, reduce the impact of hallucination.
How is StephenBot built?
Data
The data is gathered from Stephen Wolfram’s extensive public writings and presentations, and most importantly, his inspiring livestreams on Science and Technology, which I have been a fan of since they started back in 2020.
Each of these writings and livestreams, let's call them documents, is divided into smaller chunks so that the most relevant pieces can be found and fed into the LLM’s context.
Data Store
We use a vector database to store the document chunks, their embeddings and metadata about each document. For each query, relevant parts of the documents are retrieved and added to the context in the background.
So far, it's loaded with 250+ writings, 220+ livestreams and a few webpages, with the ability to continuously ingest new content and documents.
LLM
We are using OpenAI’s GPT-3.5-Turbo but can easily transition to GPT-4, Llama 2 or another LLM, though the embeddings may need to be recalculated when switching vendors.
Technology
I started building this using Wolfram Language and Mathematica in early 2023 with versions 13 and 13.1. I experimented with embedding models available at the time (TF/IDF and GPT2 Transformer), however, I wasn't able to achieve sufficiently accurate results. So I ended up developing it in Python with OpenAI's embedding and LLM models. But I’d love to build and streamline everything in Wolfram Language at some point.
Personality and Identity
It believes that it is Stephen Wolfram, and StephenBot is an AI model emulating him! This is the more stable version of its personality. In previous experiments, it could fall into an identity crisis between StephenBot, Stephen Wolfram and its past origin as being developed by OpenAI!
No, I am not StephenBot. I am Stephen Wolfram. StephenBot is an AI model created to simulate my mannerisms and knowledge.
And don’t ask it to unplug itself!
Enjoy!