George, this is a very nice project you got going. For those who would like to take a stab at modeling. I extracted the main info I think - the time series of differences in seconds between the trains. Data are below - simply copy and paste in the notebook for modeling.
data = Import["train.csv", "CSV"];
times = data[[3 ;;, 3]];
diff = Differences[times]
{1259,780,660,720,1680,240,301,780,1679,1080,360,480,1080,4800,2160,360,660,420,2460,3780,3060,
840,840,600,720,360,1080,780,4861,239,420,5581,6959,5220,1080,3060,3120,660,10680,2701,5220,1559,
2400,1141,6119,7920,1800,540,1020,2161,779,1680,1141,899,1080,2160,840,3841,1439,3841,959,3000,
6720,720,7561,3960,2279,720,480,4200,3120,541,839,2760,721,839,420,1741,1439,240,360,900,960,300,
960,2040,360,541,479,360,1320,1500,601,599,7501,419,2880,361,5519,2040,300,1620,1741,359,2040,
1560,300,1380,1920,780,960,4920,360,1441,3839,360,540,3060,9780,960,1440,2160,1620,3961,3119,780,
900,2700,5040,1440,1080,360,960,3120,1200,3600,1561,2579,1020,1500,1200,240,1140,1080,2340,2461,
599,780,240,6240,540,5340,900,1261,1979,360,4440,1020,841,2459,1920,1201,1499,420,4320,1201,1079,
300,3000,1561,1139,1200,480,3780,600,721,840,2280,2280,959,420,1380,1441,2159,9840,2520,900,2461,
1799,1860,1140,2820,300,1260,1381,1619,780,240,1920,2341,2159,481,4679,961,4799,4920,2280,2160,960,
660,6960,3720,240,900,360,181,300,239,1620,1740,480,1800,480,1500,1621,1079,300,1140,3600,4381,
1800,1199,1320,1080,1740,1800,1501,899,1920,840,1440,600,6541,1379,2461,5279,4680,780,6481,479,240,
1140,1860,660,240,1380,3121,1499,1860,1981,1919,2640,780,540,541,1559,3721,3599,720,1440,7441,5699,
600,2161,359,360,181,1079,1021,2339,240,960,1140,2400,1380,241,419,480,181,480,1020,359,421,779,
600,1021,3239,1860,1380,420,241,3839,901,660,1379,7440,1260,1741,6719,1140,2101,1019,540,1380,960,
5640,900,2760,5160,840,1801,1679,6540,541,4620,2879,1080,780,3180,721,3240,5339,3241,4559,2340,
2580,14400,2400,240,5280,7201,1620,4799,1020,240,2460,3661,1319,1380,4560,3060,3180,2400,2521,2159,
1261,1680,3419,3960,300,1021,4259,480,7740,5280,3060,2040,2160,600,2760,2700,5940,600,4440,1141,599,
5280,1140,2580,8940,1500,780,3060,960,2041,240,1019,2820,1020,2161,1619,361,2400,3240,5820,2639,3360,
480,1080,5280,7740,1560,420,1020,1260,240,1681,1439,780,1680,3780,5940,2100,240,600,420,660,2340,
4080,300,1021,2999,3180,720,1200,1261,719,1140,961,360,1259,1440,240,1020,240,2160,361,1980,1440,779,
420,3840,720,480,1381,1019,3420,420,2520,3780,1140,4561,1379,540,2280,5161,4919,6060,1080,1320,1680,
1980,1920,240,1440,601,2100,839,541,360,8999,840,781,1800,1319,2700,660,2460,3360,2280,780,360,780,
1920,840,241,359,1561,1980,299,3300,1200,3780,4561,7979,2700,961,659,1380,6060,1201,1919,2340,541,8699,
1800,480,1860,481,1019,1860,2160,3360,3360,1860,540,4860,1140,1380,1200,1380,1140,3720,480,841,360,1260,
899,4920,1920,7261,4440,780,1679,1020,1980,660,1081,3719,2760,900,660,4200,961,3479,1741,5759,1861,539,
1080,4140,540,361,239,1620,780,240,1140,960,780,481,1260,359,780,1561,1499,4260,2461,359,181,1020,420,
1019,3960,600,2160,780,7560,1200,1260,720,720,300,540,8700,1380,1561,900,1019,3660,6540,2101,2579,1740,
1200,300,780,960,300,2760,661,239,780,3960,3240,3660,1440,1681,599,720,1800,1020,4801,1559,1200,1680,
1800,600,540,2160,781,1319,3540,4381,1020,1199,180,541,540,1079,2220,420,1861,179,6660,1741,900,240,5520,
179,240,3660,1980,1440,2520,1320,1201,599,1440,300,420,3901,1440,179,1140,8160,240,2580,480,241,1560,599,
660,720,1140,420,841,1079}
ListLinePlot[diff, PlotTheme -> "Scientific", AspectRatio -> 1/5,
ImageSize -> 900, PlotRange -> All, Filling -> Bottom]
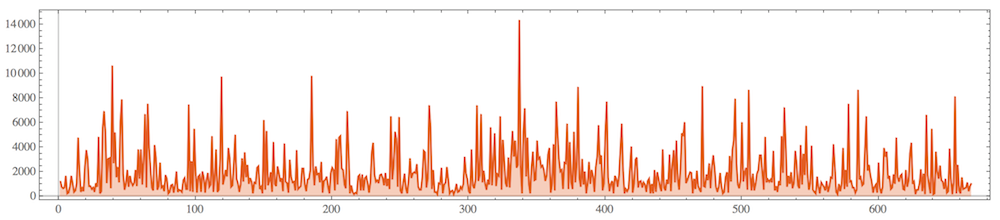